Análisis de control de calidad para detectar defectos en tornillos de sujeción en paneles de yeso
DOI:
https://doi.org/10.17981/bilo.5.1.2023.02Keywords:
Calidad en tornillos, Defectos en tornillos, Fabricación de tornillos, Tornillos para paneles de yesoAbstract
Los tornillos son piezas diseñadas para unir dos o más elementos, compuestos por tres partes principales: cabeza, cuello y rosca. Dependiendo del tipo de material del que estén hechos, su tamaño y funcionalidad pueden adquirir diferentes características. En este artículo se describe la forma en que se evaluó una muestra de 200 tornillos de 2 pulgadas utilizados para drywall con el fin de detectar posibles defectos en su proceso de fabricación, mediante la aplicación de herramientas de calidad, con lo cual se analizan los principales factores que intervienen para lograr la mejor calidad. de estos productos fueron determinados. Además, el estudio de un caso real nos permitió demostrar mejoras factibles que se pueden aplicar no solo en un área determinada, sino en toda la industria, ya que la calidad de un producto también determina el crecimiento potencial de una empresa. Los resultados obtenidos mostraron el grado de afectación de cada defecto, siendo recomendable que la empresa se enfoque en tornillos torcidos, tornillos con puntas deformadas y tornillos con medidas incorrectas, ya que estos representan mayores pérdidas. En conclusión, en la industria es necesario el uso y manejo de herramientas para incrementar la productividad y calidad de los procesos, logrando así un impacto significativo en todas las áreas de producción.
Downloads
References
E. Yildiz and F. Wörgötter, "Dcnn-based screw detection for automated disassembly processes," in 2019 15th International Conference on Signal-Image Technology & Internet-Based Systems (SITIS), 2019.
H. Gutiérrez Pulido, Calidad Total y Productividad, Mexico: McGraw-Hill/Interamericana Editores,S.A., 2010.
U. Gabler, I. Osterreicher, P. Bosk and C. Nowak, "Zero defect manufacturing as a challenge for advanced failure analysis", in 2007 IEEE/SEMI Advanced Semiconductor Manufacturing Conference, 2007.
J. Priede, "Implementation of Quality Management System ISO 9001 in the World and Its Strategic Necessity," Procedia- Social and Behavioral Sciences, vol. 58, pp. 1466-1475, 2012.
J. Breitenbach, I. Eckert, V. Mahal, H. Baumgartl, and R. Buettner, "Automated Defect Detection of Screws in the Manufacturing Industry Using Convolutional Neural Networks," from Proceedings of the 55th Hawaii International Conference on System Sciences, 2022.
S.-H. Park, K.-H. Lee, J.-S. Park and Y.-S. Shin, "Deep Learning-Based Defect Detection for Sustainable Smart Manufacturing," Sustainability, vol. 14, no. 5, p. 2697, 2022.
J. Lehr, M. Schlüter, and J. Krüger, "Classification of Similar Objects of Different Sizes Using a Reference Object by Means of Convolutional Neural Networks," from 24th IEEE International Conference on Emerging Technologies and Factory Automation (ETFA), 2019.
K. HangHong, X. JuinMing, Y. ChaoTang, and Y. JunJuh, "Screw defect detection system based on AI image recognition technology," from 2020 International Symposium on Computer, Consumer and Control (IS3C), 2020.
M. Ferhat, M. Ritou, P. Leray and N. Le-Du, "Incremental discovery of new defects: application to screwing process monitoring," Elsevier, vol. 70, no. 1, pp. 369-372, 2021.
Y. H. Hung, "Developing an Anomaly Detection System for Automatic Defective Products' Inspection," Processes, vol. 10, no. 8, p. 1476, 2022.
P. Bergmann, M. Fauser, D. Sattlegger, and C. Steger, "MVTec AD--A comprehensive real-world dataset for unsupervised anomaly detection," from Proceedings of the IEEE/CVF conference on computer vision and pattern recognition, 2019.
J. Yang, S. Li, Z. Wang, and G. Yang, "Real-Time Tiny Part Defect Detection System in Manufacturing Using Deep Learning," Opt. IEEE Access, vol. 7, pp. 89278-89291, 2019.
T. Wang, Y. Chen, M. Qiao, and H. Snoussi , "A fast and robust convolutional neural network-based defect detection model in product quality control," The International Journal of Advanced Manufacturing Technology, vol. 94, no. 9, pp. 3465-3471, 2018.
L. Song, X. Li, Y. Yang, X. Zhu, Q. Guo, and H. Yang, "Detection of Micro-Defects on Metal Screw Surfaces Based on Deep Convolutional Neural Networks," Sensors, vol. 18, no. 11, pp. 3709-3723, 2018.
M. Pour, H. Hatefnia, A. Dorieh, M. V. Kiamahalleh and Y. M. Afrouzi. M. Afrouzi, "Research on Medium density
fiberboard (MDF) behavior against screw axial withdrawal: Impact of density and operational variables," Elsevier, vol. 39, pp. 194-206, 2022.
H. Ukida, "Visual defect inspection of rotating screw heads," from SICE Annual Conference 2007, 2007.
S. Bansal, S. L. Tyagi, and V. K. Verma, "Performance Modeling and Availability Analysis of Screw Manufacturing Plant,"Materials Today: Proceedings, vol. 57, pp. 1985-1988, 2022.
P. Martinez, M. Al-Hussein and R. Ahmad, "Intelligent vision-based online inspection system of screw-fastening operations in light-gauge steel frame manufacturing," The International Journal of Advanced Manufacturing Technology, vol. 109, no. 3, pp. 645-657, 2020.
S. Yang, H. Liu, Y. Zhang, T. Arndt, C. Hofmann, B. Häfner, and G. Lanza, "A Data-Driven Approach for Quality Analytics of Screwing Processes in a Global Learning Factory," Elsevier, vol. 45, pp. 454-459, 2020.
S. C. Kiong, L. Y. Lee, S. H. Chong, M. A. Azlan, and N. H. Muhd-Nor, "Decision making with the analytical hierarchy process (AHP) for material selection in screw manufacturing for minimizing environmental impacts," from 3rd International Conference on Environmental Impact of Screw Manufacturing in the United States of America, 3rd International Conference on Environmental Impact of Screw Manufacturing in the United States of America, New York, USA. on Mechanical and Manufacturing Engineering, 2013.
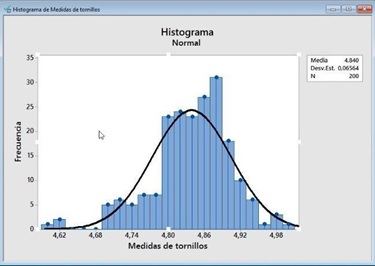