Mitigation of Nonlinear Effects using Machine Learning in Coherent Optical Access Networks
DOI:
https://doi.org/10.17981/ingecuc.17.2.2021.02Keywords:
coherent communications, digital signal processing, machine learning, optical access networks, quadrature amplitude modulationAbstract
Introduction/Context: The use of coherent detection jointly with high-level modulation formats such as 16 and 64-QAM seems to be a convenient strategy to increment capacity of future optical access networks. However, coherent detection requires high complexity digital signal processing to mitigate different impairments.
Objective: Mitigate signal distortions using nonsymmetrical demodulation techniques based on Machine Learning (ML) algorithms.
Methodology: First, a single channel Nyquist m-QAM system at 28 and 32 Gbps was simulated in VPIDesignSuite software. Then, different signals modulated at 16 and 64-QAM were generated with different laser linewidth, transmission distances and launch powers. Two ML algorithms were implemented to carry out the demodulation of the generated signals. The performance of the algorithms was evaluated using the bit error rate (BER) in terms of different system parameters as laser linewidth, transmission distance, launch power and modulation format.
Results: The use of ML allowed gains up to 2 dB in terms of optical signal-to-noise ratio at a BER value of for 16-QAM and 1.5 dB for 64-QAM. Also, the use of ML showed that it is possible to use a lower cost laser (100 kHz linewidth vs 25 kHz) with a better BER performance than using conventional demodulation.
Conclusions: We showed that the use of both algorithms could mitigate nonlinear effects and could reduce computational complexity for future optical access networks.
Downloads
References
M. Romagnoli, V. Sorianello, M. Midrio, F. H. L. Koppens, C. Huyghebaert, D. Neumaier, P. Galli, W. Templ, Antonio D’Errico & A. C. Ferrari, “Graphene-based integrated photonics for next-generation datacom and telecom,” Nat Rev Mater, vol. 3, pp. 392–414, Oct. 2018. https://doi.org/10.1038/s41578-018-0040-9
K. Shafique, B. A. Khawaja, F. Sabir, S. Qazi & M. Mustaqim, “Internet of Things (IoT) For Next-Generation Smart Systems: A Review of Current Challenges, Future Trends and Prospects for Emerging 5G-IoT Scenarios,” IEEE Access, vol. 8, pp. 1–1, 2020. https://doi.org/10.1109/access.2020.2970118
M. Zhong, Y. Yang, H. Yao, X. Fu, O. A. Dobre & O. Postolache, “5G and IoT: Towards a new era of communications and measurements,” IEEE Instrum Meas Mag, vol. 22, no. 6, pp. 18–26, Dec. 2019. https://doi.org/10.1109/MIM.2019.8917899
D. M. Marom, P. D. Colbourne, A. D’Errico, N. K. Fontaine, Y. Ikuma, R. Proietti, L. Zong, J. M. Rivas-Moscoso & I. Tomkos, “Survey of Photonic Switching Architectures and Technologies in Support of Spatially and Spectrally Flexible Optical Networking [Invited ,” J Opt Commun Netw, vol. 9, no. 1, pp. 1–, 2017. https://doi.org/10.1364/jocn.9.000001
S. Kartalopoulos & C. Qiao, “Optical access networks,” IEEE Commun Mag, vol. 43, no. 2, pp. S4–S4, Feb. 2005. https://doi.org/10.1109/MCOM.2005.1391495
L. Li, Z. Tao, L. Dou, W. Yan, S. Oda, T. Tanimura, T. Hoshida & J. C. Rasmussen, “Implementation Efficient Nonlinear Equalizer Based on Correlated Digital Backpropagation,” presented at 2011 Opt Fiber Commun Conf Expo Natl Fiber Opt Eng Conf, OFC, LA Ca. USA., 6-10 Mar. 2011. https://doi.org/10.1364/ofc.2011.oww3
L. Beygi, E. Agrell, P. Johannisson, M. Karlsson & H. Wymeersch, “The Limits of Digital Backpropagation in Nonlinear Coherent Fiber-Optical Links,” presented at 2012 38th Eur Conf Exhib Opt Commun, ECEOC, AMS, 2013. https://doi.org/10.1364/eceoc.2012.p4.14
J. Mata, I. de Miguel, R. J. Durán, N. Merayo, S. K. Singh, A. Jukan & M. Chamania, “Artificial intelligence (AI) methods in optical networks: A comprehensive surveyOpt Switch Netw, vol. 28, no.1 , pp. 43–57, Apr. 2018. https://doi.org/10.1016/j.osn.2017.12.006
Z. Dong, F. N. Khan, Q. Sui, K. Zhong, C. Lu & A. P. T. Lau, “Optical Performance Monitoring: A Review of Current and Future Technologies,” J Light Technol, vol. 34, no. 2, pp. 525–543, 15 Jan. 2016. https://doi.org/10.1109/JLT.2015.2480798
F. Musumeci, C. Rottondi, A. Nag, I. Macaluso, D. Zibar, M. Ruffini & M. Tornatore, “An Overview on Application of Machine Learning Techniques in Optical Networks,” IEEE Commun Surv Tutorials, vol. 21, no. 2, pp. 1383–1408, Sep. 2019. https://doi.org/10.1109/COMST.2018.2880039
C. Häger & H. D. Pfister, “Nonlinear interference mitigation via deep neural networks,” presented at Opt InfoBase Conf Pap, OFC, SD CA, USA, 11-15 Mar. 2018. https://doi.org/10.1364/OFC.2018.W3A.4
C. Y. Chuang, L. C. Liu, C. C. Wei, J. J. Liu, L. Henrickson, W. J. Huang, C. L. Wang, Y. K. Chen & J. Chen, “Convolutional neural network based nonlinear classifier for 112-Gbps high speed optical link,” presented at Opt InfoBase Conf Pap, OFC, SA, CA, USA, 11-15 Mar. 2018. https://doi.org/10.1364/OFC.2018.W2A.43
S. Liu, Y. M. Alfadhli, S. Shen, H. Tian & G. K. Chang, “Mitigation of multi-user access impairments in 5G A-RoF-based mobile fronthaul utilizing machine learning for an artificial neural network nonlinear equalizer2018 Opt Fiber Commun Conf Expo OFC 2018 - Proc, OFC, SA, CA, USA, 11-15 Mar. 2018. Available: https://ieeexplore.ieee.org/document/8386151
D. Wang, M. Zhang, Z. Cai, Y. Cui, Z. Li, H. Han, M. Fu & B. Luo, “Combatting nonlinear phase noise in coherent optical systems with an optimized decision processor based on machine learning,” Opt Commun, vol. 369, no. 1, pp. 199–208, Jun. 2016. https://doi.org/10.1016/j.optcom.2016.02.029
L. Sun, J. Du, G. Chen, Z. He, X. Chen & G. T. Reed, “Machine-learning detector based on support vector machine for 122-Gbps multi-CAP optical communication system,” presented at 2017 Opto-Electronics Commun Conf OECC 2017 Photonics Glob Conf PGC 2017, OECC, SG, 31 Jul.-4 Aug. 2017. https://doi.org/10.1109/OECC.2017.8114893
E. A. Fernández, J. J. G. Torres, A. M. C. Soto & N. G. Gonzalez, “Radio-over-Fiber signal demodulation in the presence of non-Gaussian distortions based on subregion constellation processing,” Opt Fiber Technol, vol. 53, no. 1, pp. 102062, Dec. 2019. https://doi.org/10.1016/j.yofte.2019.102062
H. Nain, U. Jadon & V. Mishra, “Evaluation and analysis of non-linear effect in WDM optical network,” IEEE Int Conf Recent Trends Electron Inf Commun Technol RTEICT 2016 - Proc, RTEICT, BLR, IMD, 20-21 May. 2017. https://doi.org/10.1109/RTEICT.2016.7807777
T. Okamoto & F. Ito, “Laser Phase Noise Characterization Using Parallel Linear Optical Sampling,” J Lightwave Technol, vol. 32, no. 18, pp. 3119–3125, 2014. Available: https://www.osapublishing.org/jlt/abstract.cfm?uri=jlt-32-18-3119
R.-J. Essiambre, G. Kramer, P. J. Winzer, G. J. Foschini & B. Goebel, “Capacity Limits of Optical Fiber Networks PART 1: DIGITIZATION, CAPACITY, AND CONSTELLATIONS,” J Light Technol, vol. 28, no. 4, pp. 662–701, 15 Feb. 2010. https://doi.org/10.1109/JLT.2009.2039464
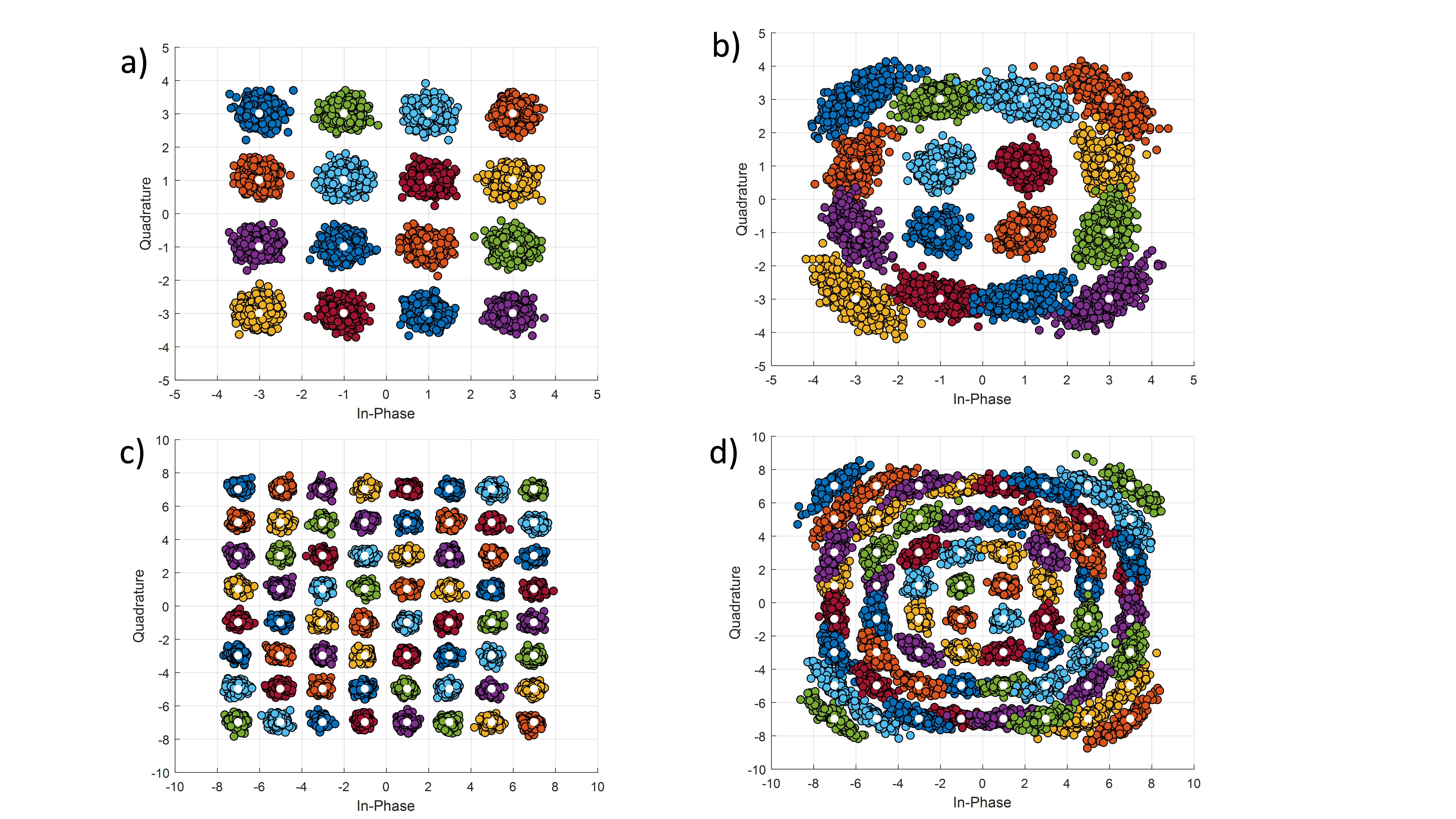
Published
How to Cite
Issue
Section
License
Copyright (c) 2021 INGE CUC

This work is licensed under a Creative Commons Attribution-NonCommercial-NoDerivatives 4.0 International License.
Published papers are the exclusive responsibility of their authors and do not necessary reflect the opinions of the editorial committee.
INGE CUC Journal respects the moral rights of its authors, whom must cede the editorial committee the patrimonial rights of the published material. In turn, the authors inform that the current work is unpublished and has not been previously published.
All articles are licensed under a Creative Commons Attribution-NonCommercial-NoDerivatives 4.0 International License.