Cluster-RNA Method to Classify, Characterize and Predict Competitive Profiles of the Retail Stores Sector in the City of Barranquilla
DOI:
https://doi.org/10.17981/ingecuc.16.1.2020.17Keywords:
cluster analysis, artificial neural network, retailAbstract
Objective– To develop a method to classify, characterize and forecast competitive profiles of the retail stores sector based on the integration of the cluster analysis technique and artificial neural networks.
Methodology– For the above, the literature related to the competitiveness of retail stores was reviewed, from which variables associated with this research were identified. The information analyzed corresponds to 224 retail stores in the city of Barranquilla.
Results– The cluster analysis allowed to characterize 4 competitive profiles of the sector that showed to be homogeneous intragroup and heterogeneous extragroup. The artificial neural network model showed a 91.3% correct classification in the reserve sample, which inferred the capacity of classification of the network model and the discriminant capacity of the variables related to the knowledge of products and prices, the practices of inventory and sales, presence in the market, differentiated attention, location and variety of products in the identified profiles.
Conclusions– The results of the research show high capacity of the cluster-RNA method, to classify and project competitive profiles from which improvement processes can be designed.
Downloads
References
E. L. Aldana, “Imagen y Posicionamiento de las Tiendas de Barrio en Colombia, aplicando el análisis factorial de correspondencias AFC”, Panorama Económico U. de C., vol. 225, no. 15, pp. 255–281, Jan. 2015. Disponible en http://hdl.handle.net/11227/3742
J. Córdoba & L. Cano, “Estudio de hábitos y valores de los consumidores del canal tradicional tiendas de barrio de la ciudad de Cali”, Entramado, vol. 5, no. 2, pp. 44–63, Dec. 2009. Disponible en https://revistas.unilibre.edu.co/index.php/entramado/article/view/3354
C. Martínez & M. Vergara, “Marketing relacional y su contribución a la competitividad en las tiendas de barrio del sur oriente de Barranquilla”. M.S. Thesis, UAC, BQ, CO, 2018.
Q. Martin, M. Cabero & Y. De Paz, Tratamiento Estadístico de Datos con SPSS. Madrid, ES: Thomson Editores, pp. 145–156, 2008.
D. Peña, Análisis de datos multivariantes. Madrid, ES: McGraw Hill, pp. 137–235, 2002.
M. Ato, J. López, A. Velandrino & J. Sánchez, Estadística Avanzada con el paquete Systat. Murcia, ES: UMU, 1990.
P. Arroyo & J. Borja, Análisis Multivariante para la inteligencia de Mercados. Monterrey, MX: Editorial Digital del ITESM, 2018.
J. De la Garza, B. Morales & B. González, Análisis Estadístico Multivariante. Un enfoque teórico y práctico. México, D.F. MX: McGraw Hill, 2013.
A. Catena, M. Ramos & H. Trujillo, Análisis Multivariado. Un manual para investigadores (Primera). Madrid, ES: Editorial Biblioteca Nueva, 2003.
H. Alaka, L. Oyedele, H. Owolabi, V. Kumar, S. Ajayie, O. Akinade & M. Bilalf, “Systematic Review of Bankruptcy Prediction Models: Towards a Framework for Tool Selection”, Expert Syst. with Appl., vol. 94, pp. 164–184, Mar. 2018. https://doi.org/10.1016/j.eswa.2017.10.040
G. McMillan, Process/Industrial Instruments and Controls Handbook. USA: McGraw Hill, 2013.
O. García & A. Morales, “Desempeño financiero de las empresas: una propuesta de clasificación por RNA”. Dimensión Empresarial, vol. 14, no. 2, pp. 11–23. 2016. https://dx.doi.org/10.15665/rde.v14i2.686
L. Álvarez, Fundamentos de inteligencia artificial. Murcia, ES: EDITUM, 1994.
R. Flórez & J. Fernández, “Las Redes Neuronales Artificiales. Fundamentos teóricos y aplicaciones prácticas”. Madrid, ES: Netbiblo, 2008.
C. Pérez, Redes Neuronales a través de ejemplos. Aplicaciones con Matlab. Scotts Valley, USA: CreateSpace Independent Publishing Platform, 2017.
E. Caicedo & J. López, Una Aproximación Práctica a las Redes Neuronales Artificiales (Primera). Cali, CO: Programa Editorial Univalle, 2009. https://doi.org/10.25100/peu.64
A. Ong, B. Uchino & E. Wethington, “Loneliness and health in older adults: A mini-review and synthesis”, Gerontology, vol. 62, pp. 4434–4449, Jun. 2016. https://doi.org/10.1159/000441651
E. De la Hoz & L. López, “Aplicación de Técnicas de Análisis de Conglomerados y Redes Neuronales Artificiales en la Evaluación del Potencial Exportador de una Empresa”. Inf. Tecnol., vol. 28, no. 4, pp. 67–74, Jan. 2017. https://doi.org/10.4067/S0718-07642017000400009
E. De La Hoz, T. Fontalvo & L. López, “Análisis Envolvente de Datos y Cálculo Multivariado para Valorar, Clasificar y Predecir la Eficiencia Productiva y de Innovación de las Empresas del Sector Químico”, Inf. Tecnol, vol. 30, no. 5, pp. 213–220, Oct. 2019. https://doi.org/10.4067/S0718-07642019000500213
E. De la Hoz, J. Morelos & T. Fontalvo, “Methodology with multivariate calculation to define and evaluate financial productivity profiles of the chemical sector in Colombia”, IJPQM, vol. 27, no. 2, pp. 144–160, Jun. 2019. https://doi.org/10.1504/IJPQM.2019.100141
E. De la Hoz, J. Morelos & L. López, “Evaluación de la competitividad comercial del sector industrial colombiano mediante el coeficiente de apertura exportadora”, Aglala, vol. 10, no. 1, pp. 180–195, 2019. Disponible en https://revistas.curn.edu.co/index.php/aglala/article/view/1343
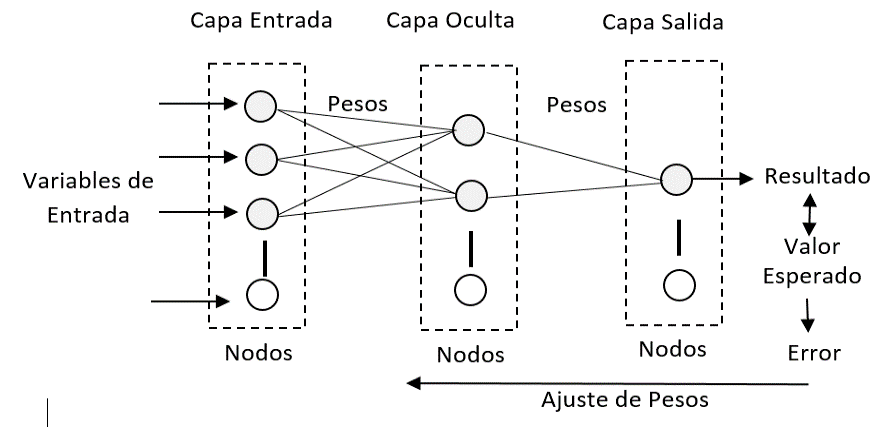
Published
How to Cite
Issue
Section
License
Copyright (c) 2020 INGE CUC

This work is licensed under a Creative Commons Attribution-NonCommercial-NoDerivatives 4.0 International License.
Published papers are the exclusive responsibility of their authors and do not necessary reflect the opinions of the editorial committee.
INGE CUC Journal respects the moral rights of its authors, whom must cede the editorial committee the patrimonial rights of the published material. In turn, the authors inform that the current work is unpublished and has not been previously published.
All articles are licensed under a Creative Commons Attribution-NonCommercial-NoDerivatives 4.0 International License.