Implementation of the VNS-DEEPSO algorithm for the energy dispatch in smart distributed grid
DOI:
https://doi.org/10.17981/ingecuc.15.1.2019.13Keywords:
heuristic algorithms, renewable energy, optimization, intelligent network, electric vehiclesAbstract
Introduction: Traditional electric networks are migrating to new configurations of intelligent networks, which bring operational and planning challenges. In order to advance in these challenges, an optimization problem is proposed to solve in the programming of intelligent network elements.
Objective: The optimization problem consists of managing the energy dispatch of an intelligent network to optimize the available resources, considering the uncertainty of renewable energies, planned trips of electric vehicles, cargo forecast and market prices.
Methodology: It was proposed to use an assembly between two heuristic methods. The VNS algorithm (Variable Neighborhood Search) and the DEEPSO (Differential Evolutionary Particle Swarm).
Results: The value obtained by the VNS-DEEPSO algorithm was 18.21, being 7 % better than the second algorithm classified in the competition.
Conclusions: The VNS-DEEPSO algorithm was the winner among 9 metaheuristic algorithms that solved the problem. This problem has a greater increase in difficulty due to the uncertainty generated by weather conditions, load forecast, planned EV´s trips, and market prices. According to the results, the VNS-DEEPSO algorithm proved to be the most efficient in minimizing operational costs and maximizing the revenues of the intelligent network.
Downloads
References
M. Tuballa and M. Abundo, “A review of the development of Smart Grid technologies,” Renewable and Sustainable Energy Reviews, vol. 59, no. 1, pp. 710–725, Jun. 2016. Doi: https://doi.org/10.1016/j.rser.2016.01.011
F. Lezama, J. Soares, Z. Bale, J. Rueda, S. Rivera and I. Elrich, “2017 IEEE competition on modern heuristic optimizers for smart grid operation: Testbeds and results,” Swarm and Evolutionary Computation, vol. 44, no. 1, pp. 420–427, Feb. 2019. Doi: https://doi.org/10.1016/j.swevo.2018.05.005
O. Dzobo, A. M. Shehata and C. L Azimoh, “Optimal economic load dispatch in smart grids considering uncertainty”. In AFRICON, 2017 IEEE., Cape Town, South Africa, Sept. 18–20, 2019, pp. 1277–1282. Doi: https://doi.org/10.1109/AFRCON.2017.8095666
F. Lezama, J. Soares, Z. Bale and J. Rueda, “WCCI 2018 Competition Evolutionary Computation in Uncertain Environments: A Smart Grid Application. Test bed: Optimal scheduling of distributed energy resources considering uncertainty of renewables, EVs, load forecast and market prices,” in Proc. IEEE WCCI, Rio de Janeiro, Brazil, Jul. 8–13, 2018, pp. 1–19. Available in: http://www.gecad.isep.ipp.pt/WCCI2018-SG-COMPETITION/
Y. Jin and J. Branke, “Evolutionary Optimization in Uncertain Environments—A Survey,” IEEE Trans. Evol. Comput., vol. 9, no. 3. pp. 303–317, Jun. 2005. Doi: https://doi.org/10.1109/TEVC.2005. 846356
M. Gendreau and J.-Y. Potvin, “Handbook of Metaheuristics,” vol. 2, Switzerland: Springer Science+Business Media, 2010. Doi: https://doi.org/10.1007/978-1-4419-1665-5
Y. Zhao, J. Cao, X. Lai, C. Yin and T. Chen, “Ensemble based constrained-optimization extreme learning machine for landmark recognition,” 2015 34th Chinese Control Conference (CCC), Hangzhou, China, 28–30 Jul. 2015, pp. 3884–3889. Doi: https://doi.org/10.1109/ChiCC.2015.7260239
S. Hui and P. N. Suganthan, “Ensemble and Arithmetic Recombination-Based Speciation Differential Evolution for Multimodal Optimization,” in IEEE Transactions on Cybernetics, vol. 46, no. 1, pp. 64–74, Jan. 2016. Doi: https://doi.org/10.1109/TCYB.2015.2394466
X. Li, S. Ma and Y. Wang, “Multi-Population Based Ensemble Mutation Method for Single Objective Bilevel Optimization Problem,” in IEEE Access, vol. 4, no. 1, pp. 7262–7274, Oct. 2016. Doi: https://doi.org/10.1109/ACCESS.2016.2617738
V. Miranda and R. Alves, “Differential Evolutionary Particle Swarm Optimization (DEEPSO): A Successful Hybrid,” 2013 BRICS Congress on Computational Intelligence and 11th Brazilian Congress on Computational Intelligence, Ipojuca, Brazil, 8–11 Sept. 2013, pp. 368–374. Doi: https://doi.org/10.1109/BRICS-CCI-CBIC.2013.68
D. G. Mayer, B. P. Kinghorn and A. A. Archer, “Differential evolution – an easy and efficient evolutionary algorithm for model optimisation,” Agricultural Systems, vol 83, no. 3, pp. 315–328, Mar. 2005. Doi: https://doi.org/10.1016/j.agsy.2004.05.002
F. Marini and B. Walczak, “Particle swarm optimization (PSO). A tutorial,” Chemometrics and Intelligent Laboratory Systems, vol 149, Part B, pp. 153–165, Dec. 2015. Doi: https://doi.org/10.1016/j.chemolab.2015.08.020
P. Hansen and N. Mladenović, “Variable neighborhood search: Principles and applications,” European Journal of Operational Research, vol. 130, no. 3, pp. 449–467, May. 2001. Doi: https://doi.org/10.1016/S0377-2217(00)00100-4
E. Vargas, L. Macedo, P. Bueno and R. Romero, “A VNS algorithm for the design of supplementary damping controllers for small-signal stability analysis,” International Journal of Electrical Power & Energy Systems, vol. 94, no. 1, pp. 41–56, Jan. 2018. Doi: https://doi.org/10.1016/j.ijepes.2017.06.017
R. Brandl, P. Kotsampopoulos, G. Lauss, M. Maniatopoulos, M. Nuschke, J. Montoya and D. Strauss-Mincu, “Advanced Testing Chain Supporting the Validation of Smart Grid Systems and Technologies,” in 2018 IEEE Workshop on Complexity in Engineering, Florence, Italy, Oct. 10–12, 2018. pp. 1–6. Doi: https://doi.org/10.1109/CompEng.2018.8536223
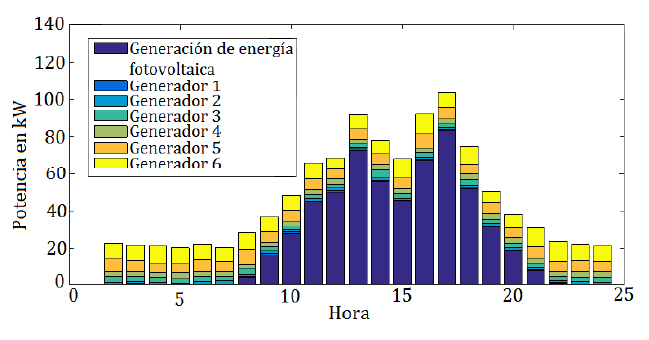
Published
How to Cite
Issue
Section
License
Copyright (c) 2019 INGE CUC

This work is licensed under a Creative Commons Attribution-NonCommercial-NoDerivatives 4.0 International License.
Published papers are the exclusive responsibility of their authors and do not necessary reflect the opinions of the editorial committee.
INGE CUC Journal respects the moral rights of its authors, whom must cede the editorial committee the patrimonial rights of the published material. In turn, the authors inform that the current work is unpublished and has not been previously published.
All articles are licensed under a Creative Commons Attribution-NonCommercial-NoDerivatives 4.0 International License.