Anti-collision system for blind people using evolutionary neural networks
DOI:
https://doi.org/10.17981/ingecuc.14.2.2018.03Keywords:
Cooperative co-evolutionary genetic algorithm, blind, anti-collision system, neuro-evolutionary method, artificial neural networkAbstract
Introduction: This paper shows the design and implementation of an anti-collision system for blind people using evolutionary artificial neural networks (EANNs).
Objective: Present the implementation of evolutionary neural networks in a guide system for the blind in the detection of static and moving obstacles.
Method: Methodology is based on the creation of artificial neural networks from the cooperative co-evolutionary genetic algorithm (CCGA), which is responsible for structuring, modifying and training neural networks. It uses the network definition matrix (NDM). NDM is made of a chromosome "chromosome is genetic algorithm part" is taken as the basis. Once is done the NDM, it creates an artificial neural network to be trained.
Results: Program carried out several neural networks, and generated 10 chromosomes in each execution. Artificial neural networks were trained with the CCGA and it applies the cooperation, they obtained the best anti-collision neural networks considering a definite time, anti-collision neural networks worked effectively for the detection of physical obstacles and with movement.
Conclusions: In the anti-collision system for the blind, we observed the effectiveness of neural networks in giving an answer, detecting both static and moving objects, providing security to the blind, avoiding collisions with objects.
Downloads
References
J. Mosquera and D. Rodríguez, "Sistema de reconocimiento de obstáculos para movilidad de Invidentes," tesis de pregrado, Universidad del Cauca, 2014. [2] T. Praczyk, "Neural anti-collision system for autonomous surface vehicle," Neurocomputing, vol. 149, pp. 559–572, 2015. https://doi.org/10.1016/j.neucom.2014.08.018
D. Curran and C. O'Riordan, "Increasing population diversity through cultural learning," Adaptive Behavior, vol. 14, no. 4, pp. 315–338, 2006. https://doi.org/10.1177/1059712306072335
G. Miller, P. Todd, and S. Hegde, "Designing neural networks using genetic algorithms," Proceedings of the 3rd International Conference on Genetic Algorithms, pp. 379–384, 1989.
X. Yao and Y. Liu, "A new evolutionary system for evolving artificial neural networks," IEEE transactions on neural networks, vol. 8, no. 3, pp. 694–713, 1997. https://doi.org/10.1109/72.572107
N. García, C. Hervás, and J. Mu-oz, "Multi-objective cooperative coevolution of artificial neural networks," Neural Networks, vol. 15, no. 10, pp. 1259–1278, 2002. https://doi.org/10.1016/S0893-6080(02)00095-3
K. Ohkura, T. Yasuda, Y. Kawamatsu, Y. Matsumura, and K. Ueda, "MBEANN: Mutation-based evolving artificial neural networks," Neural Networks, pp. 936–945, 2007. https://doi.org/10.1007/978-3-540-74913-4_94
A. Azzini and A. Tettamanzi, "A new genetic approach for neural network design and optimization," tesis doctoral, Universidad de Milán, 2008.
A. Tallón, "Nuevos modelos de redes neuronales evolutivas para clasificación: aplicación a unidades producto y unidades sigmoide," tesis doctoral, Universidad de Sevilla, 2013.
A. Carvalho, "A cooperative coevolutionary genetic algorithm for learning bayesian network structures," Proceedings of the 13th Annual Genetic and Evolutionary Computation Conference, no. 1, pp. 1131–1138, 2011. https://doi.org/10.1145/2001576.2001729
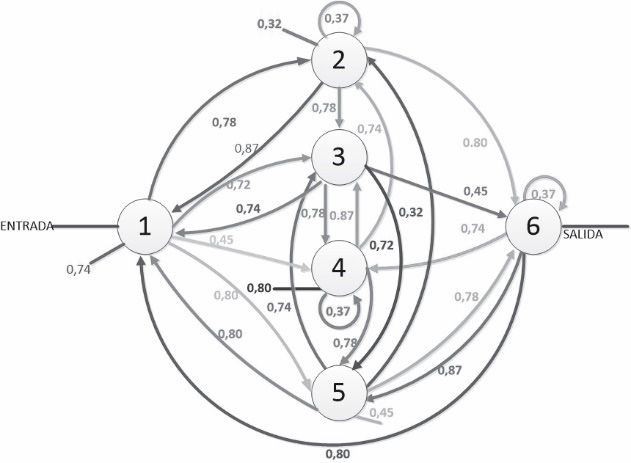
Downloads
Published
How to Cite
Issue
Section
License
Published papers are the exclusive responsibility of their authors and do not necessary reflect the opinions of the editorial committee.
INGE CUC Journal respects the moral rights of its authors, whom must cede the editorial committee the patrimonial rights of the published material. In turn, the authors inform that the current work is unpublished and has not been previously published.
All articles are licensed under a Creative Commons Attribution-NonCommercial-NoDerivatives 4.0 International License.