Técnicas de Machine Learning aplicadas al consumo de sustancias psicoactivas ilícitas: Un mapeo sistémico
DOI:
https://doi.org/10.17981/ingecuc.19.2.2023.08Palabras clave:
Machine learning, sustancias psicoactivas ilegales,, drogas ilegales, modelos predictivos, nuevas sustancias psicoactivas ilegalesResumen
Introducción— El consumo de sustancias psicoactivas ilícitas es una problemática que se vive a diario, donde personas de diferentes edades se han visto implicadas, resaltando que muchas de estas sustancias generan trastornos tales como, por ejemplo: la Marihuana o cannabis: su consumo afecta la función cerebral de manera directa, y particularmente las partes del cerebro responsables de la memoria, el aprendizaje, la atención, la toma de decisiones. El Bazuco: es una sustancia tóxica, cuyos principales riesgos de consumirla se reflejan en el deterioro neurológico y en el organismo, y es muy rápida su disolución en el torrente sanguíneo, aspecto que hace que sea muy adictiva. La Cocaína: su consumo afecta directamente el sistema nervioso y el resto del organismo de forma inmediata, en estas afectaciones se encuentran vasoconstricción, midriasis, hipertermia, taquicardia e hipertensión. La Heroína: es una sustancia altamente adictiva, inicialmente, sus efectos son muy placenteros, lo que propicia una conducta de consumo continuada y repetitiva, además, produce sensaciones de sequedad en la boca, enrojecimiento y acaloramiento de la piel, pesadez en brazos y piernas, náuseas y vómitos, comezón intensa y enturbiamiento de las facultades mentales.
Objetivo— Esta problemática es algo que resalta mucho y de gran impacto en los jóvenes de acuerdo al contexto en el que se encuentren ya que hoy en dia hay mucha facilidad para obtener este tipo de sustancias, por ende, se han planteado una serie de trabajos que abordan desde la inteligencia artificial esa problemática.
Metodología— El presente estudio realiza una revisión de 50 publicaciones relacionadas con el uso de métodos y técnicas de ML aplicadas al consumo de sustancias psicoactivas ilícitas.
Resultados— De las publicaciones incluidas se hallaron temáticas en común por lo que se hace un resumen de los artículos seleccionados por cada temática y se describen brevemente los métodos adoptados, así como también una comparativa entre ellos, anotando los métodos usados, sus resultados y demás factores importantes de la aplicación o modelo en distintas áreas y se concluye con una serie de propuestas sobre las líneas que a futuro podrían encaminar la investigación en este campo.
Descargas
Citas
PAHO. “Abuso de sustancias”, (Consultado en mayo 2, 2023). [En línea . Disponible en https://www.paho.org/es/temas/abuso-sustancias
ONU. “Comunicado de prensa. El Informe Mundial sobre las Drogas 2022 de la UNODC destaca las tendencias del cannabis posteriores a su legalización, el impacto ambiental de las drogas ilícitas y el consumo de drogas entre las mujeres y las personas jóvenes”, junio 27, 2022. [En línea. Disponible en https://www.unodc.org/unodc/es/press/releases/2022/June/unodc-world-drug-report-2022-highlights-trends-on-cannabis-post-legalization--environmental-impacts-of-illicit-drugs--and-drug-use-among-women-and-youth.html
MinSalud, Política Integral para la Prevención y Atención del Consumo de Sustancias Psicoactivas. BO, CO: MinSalud, 2019. Recuperado de https://www.minsalud.gov.co/sites/rid/Lists/BibliotecaDigital/RIDE/VS/PP/politica-prevencion-atencion-spa.pdf
H. Zhou, J. Tang & H. Zheng, “Editorial Machine Learning for Medical Applications,” Sci. World J., pp. 1–2, Nov. 2014. https://doi.org/10.1155/2015/825267
D. Carrizo y J. Rojas, “Clasificación de prácticas de educción de requisitos en desarrollos ágiles: un mapeo sistemático”, Ingeniare Rev. Chilena Ing., vol. 24, no. 4, pp. 654–662, Mar. 2016. http://dx.doi.org/10.4067/S0718-33052016000400010
B. Kitchenham, “Procedures for Performing Systematic Reviews,” KEE, UK: KU, Joint Technical Report, 2004. Available from https://www.inf.ufsc.br/~aldo.vw/kitchenham.pdf
X. Gu, B. Yang, S. Gao, L. Yan, D. Xu & W. Wang, “Application of bi-modal signal in the classification and recognition of drug addiction degree based on machine learning,” Math. Biosci. Eng., vol. 18, no. 5, pp. 6926– 6940, Aug. 2021. https://doi.org/10.3934/MBE.2021344
M. Hassan, Z. Peya, S. Zaman, J. Angon, A. Keya & A. Dulla, “A Machine Learning Approach to Identify the Correlation and Association among the Students' Drug Addict Behavior,” presented at 11th International Conference on Computing, Communication and Networking Technologies, ICCCNT, KGR, IN, 1-3 Jul. 2020. https://doi.org/10.1109/ICCCNT49239.2020.9225355
M. Nesa, T. Shaha & Y. Yoon, “Prediction of juvenile crime in Bangladesh due to drug addiction using machine learning and explainable AI techniques,” J. Comput. Soc. Sci., vol. 5, no. 2, pp. 1467–1487, Aug. 2022. https://doi.org/10.1007/s42001-022-00175-7
A. Arif, S. Sany, F. Sharmin, S. Rahman & T. Habib, “Prediction of addiction to drugs and alcohol using machine learning: A case study on Bangladeshi population,” Int. J. Electr. Comput. Eng., vol. 11, no. 5, pp. 4471–4480, Mar. 2021. https://doi.org/10.11591/ijece.v11i5.pp4471-4480
H. Gong, C. Xie, C. Yu, N. Sun, H. Lu & Y. Xie, “Psychosocial factors predict the level of substance craving of people with drug addiction: A machine learning approach,” Int. J. Environ. Res. Public Health, vol. 18, no. 22, pp. 1–12, Nov. 2021. https://doi.org/10.3390/ijerph182212175
U. Islam, E. Haque, D. Alsalman, M. Islam, M. Moni & I. Sarker, “A Machine Learning Model for Predicting Individual Substance Abuse with Associated Risk-Factors,” Ann. Data Sci., pp. 1–28, Mar. 2022. https://doi.org/10.1007/s40745-022-00381-0
N. Zulkifli, Z. Cob, A. Latif & S. Drus, “A Systematic Review of Machine Learning in Substance Addiction,” in 8th International Conference on Information Technology and Multimedia, ICIMU, SLR, MY, 24-26 Aug. 2020. https://doi.org/10.1109/ICIMU49871.2020.9243581
J. Mollick & H. Kober, “Computational models of drug use and addiction: A review,” J. Abnorm. Psychol., vol. 129, no. 6, pp. 544–555, Aug. 2020. https://doi.org/10.1037/abn0000503
Y. Choi & Y. Boo, “Comparing logistic regression models with alternative machine learning methods to predict the risk of drug intoxication mortality,” Int. J. Environ. Res. Public Health, vol. 17, no. 3, pp. 1–10, Jan. 2020. https://doi.org/10.3390/ijerph17030897
K. Mak, K. Lee & C. Park, “Applications of machine learning in addiction studies: A systematic review,” Psychiat. Res., vol. 275, pp. 53–60, May. 2019. https://doi.org/10.1016/j.psychres.2019.03.001
B. Chhetri, L. Goyal & M. Mittal, “How machine learning is used to study addiction in digital healthcare: A systematic review,” Int. J. Inf. Manag. Data Insights, vol. 3, no. 2, pp. 1–17, Mar. 2023. https://doi.org/10.1016/j.jjimei.2023.100175
E. Barenholtz, N. Fitzgerald & W. Hahn, “Machine-learning approaches to substance-abuse research: emerging trends and their implications,” Curr. Opin. Psychiatry, vol. 33, no. 4, pp. 334–342, Jul. 2020. https://doi.org/10.1097/yco.0000000000000611
A. Anggrawan, C. Satria, C. Nuraini, Lusiana, N. Ayu Dasriani & Mayadi, “Machine Learning for Diagnosing Drug Users and Types of Drugs Used”, IJACSA, vol. 12, no. 11, pp. 111–118, Dec. 2021. http://dx.doi.org/10.14569/IJACSA.2021.0121113
R. Vunikili, B. Glicksberg, K. Johnson, J. Dudley, L. Subramanian & K. Shameer, “Predictive Modelling of Susceptibility to Substance Abuse, Mortality and Drug-Drug Interactions in Opioid Patients,” Front. Artif. Intell., vol. 4, pp. 1–10, Oct. 2021. https://doi.org/10.3389/frai.2021.742723
B. Rekabdar, D. Albright, J. McDaniel, S. Talafha & H. Jeong, “From machine learning to deep learning: A comprehensive study of alcohol and drug use disorder,” Healthcare Anal., vol. 2, pp. 1–18, Aug. 2022. https://doi.org/10.1016/j.health.2022.100104
Y. Jing, Z. Hu, P. Fan, Y. Xue, L. Wang, R. Tarter, L. Kirisci, J. Wang, M. Vanyukov & X. Xie, “Analysis of substance use and its outcomes by machine learning I. Childhood Evaluation of Liability to Substance Use Disorder,” Drug Alcohol Depend., vol. 206, no. 3, pp. 1–16, Oct. 2019. https://doi.org/10.1016/j.drugalcdep.2019.107605
Z. Hu, Y. Jing, Y. Xue, P. Fan, L. Wang, M. Vanyukov, L. Kirisci, J. Wang, R. Tarter & X-Q. Xie, “Analysis of substance use and its outcomes by machine learning: II. Derivation and prediction of the trajectory of substance use severity,” Drug Alcohol Depend., vol. 206, pp. 1–10, Jan. 2020. https://doi.org/10.1016/j.drugalcdep.2019.107604
U. Islam, I. Sarker, E. Haque & M. Hoque, “Predicting Individual Substance Abuse Vulnerability using Machine Learning Techniques”, in Hybrid Intelligent Systems, A. Abraham, T. Hanne, O. Castillo, N. Gandhi, T. Rios & T-P.Hong, Eds., CHM, CH: Springer, 2021, pp. 412–421. https://doi.org/10.1007/978-3-030-73050-5_42
Y. Yuan, J. Huang & K. Yan, “Virtual Reality Therapy and Machine Learning Techniques in Drug Addiction Treatment,” presented at 10th International Conference on Information Technology in Medicine and Education, ITME, QGO, CN, 23-25 Aug. 2019. https://doi.org/10.1109/ITME.2019.00062
M. Hasan, G. Young, J. Shi, P. Mohite, L. Young, S. Weiner & Noor-E-Alam, “A machine learning based two- stage clinical decision support system for predicting patients’ discontinuation from opioid use disorder treatment: retrospective observational study,” BMC Med. Inform. Decis. Mak., vol. 21, no. 1, pp. 1–21, Nov. 2021. https://doi.org/10.1186/s12911-021-01692-7
J. Tapia-Galisteo, J. Iniesta, C. Perez-Gandía, G. Garcia-Saez, D. Urgelés, F. Izquierdo & M. Hernando, “Prediction of Cocaine Inpatient Treatment Success Using Machine Learning on High-Dimensional Heterogeneous Data,” IEEE Acc., vol. 8, pp. 218936–218953, Dec. 2020. https://doi.org/10.1109/ACCESS.2020.3041895
M. Symons, G. Feeney, M. Gallagher, R. Young & J. Connor, “Machine learning vs addiction therapists: A pilot study predicting alcohol dependence treatment outcome from patient data in behavior therapy with adjunctive medication,” J. Subst. Abuse Treat., vol. 99, pp. 156–162, Jan. 2019. https://doi.org/10.1016/j.jsat.2019.01.020
M. Nasir, N. Summerfield, A. Oztekin, M. Knight, L. Ackerson & S. Carreiro, “Machine learning-based outcome prediction and novel hypotheses generation for substance use disorder treatment,” J. Am. Med. Inform. Assoc., vol. 28, no. 6, pp. 1216–1224, Feb. 2021. https://doi.org/10.1093/jamia/ocaa350
S. Yip, B. Kiluk & D. Scheinost, “Toward Addiction Prediction: An Overview of Cross-Validated Predictive Modeling Findings and Considerations for Future Neuroimaging Research,” Biol. Psychiatry: Cogn. Neurosci.Neuroimaging, vol. 5, no. 8, pp. 748–758, Aug. 2020. https://doi.org/10.1016/j.bpsc.2019.11.001
J. Tapia, “Propuesta de modelos predictivos en salud mental para la personalización de terapias de rehabilitación en pacientes con adicciones,” Tesis Doctoral, ETSIT, UPM, MD, ES, 2021. Disponible en https://oa.upm.es/68120/
Y. Kobayashi & K. Yoshida, “Automated retention time prediction of new psychoactive substances in gas chromatography,” Proc. Comp. Sci., vol. 207, pp. 654–663, Sept. 2022. https://doi.org/10.1016/j.procs.2022.09.120
J. Klingberg, B. Keen, A. Cawley, D. Pasin & S. Fu, “Developments in high-resolution mass spectrometric analyses of new psychoactive substances,” Arch. Toxicol., vol. 96, no. 4, pp. 949–967, Feb. 2022. https://doi.org/10.1007/s00204-022-03224-2
E. Olesti, I. De Toma, J. Ramaekers, T. Brunt, M. Carbó, C. Fernández-Avilés, P. Robledo, M. Farré, M. Dierssen, Ó. Pozo y R. De La Torre, “Metabolomics predicts the pharmacological profile of new psychoactive substances,” J. Psychopharmacol., vol. 33, no. 3, pp. 347–354, Nov. 2018. https://doi.org/10.1177/0269881118812103
S. Wong, L. Ng, J. Tan & J. Pan, “Screening unknown novel psychoactive substances using GC–MS based machine learning,” Forensic Chem., vol. 34, pp. 1–22, Nov. 2022. https://doi.org/10.1016/j.forc.2023.100499
M. Han, S. Liu, D. Zhang, R. Zhang, D. Liu, H. Xing, D. Sun, L. Gong, P. Cai, W. Tu, J. Chen & Q-N. Hu, “AddictedChem: A Data-Driven Integrated Platform for New Psychoactive Substance Identification,” Mol., vol. 27, no. 12, pp. 1–15, Jun. 2022. https://doi.org/10.3390/molecules27123931
J. Li, Q. Xu, N. Shah & T. Mackey, “A machine learning approach for the detection and characterization of illicit drug dealers on instagram: Model evaluation study,” J. Med. Internet Res., vol. 21, no. 6, pp. 1–14, Feb. 2019. https://doi.org/10.2196/13803
S. Hassanpour, N. Tomita, T. DeLise, B. Crosier & L. Marsch, “Identifying substance use risk based on deep neural networks and Instagram social media data,” Neuropsychopharmacol., vol. 44, no. 3, pp. 487–494, Oct. 2018. https://doi.org/10.1038/s41386-018-0247-x
U. Kursuncu, M. Gaur, U. Lokala, A. Illendula, K. Thirunarayan, R. Daniulaityte, A. Sheth & I. Arpinar, “What’s ur Type? Contextualized Classification of User Types in Marijuana-Related Communications Using Compositional Multiview Embedding,” presented at International Conference on Web Intelligence, ACM/IEEE/WIC, STG, CL, 3-6 Dec. 2018. https://doi.org/10.1109/WI.2018.00-50
T. Mackey, J. Kalyanam, J. Klugman, E. Kuzmenko & R. Gupta, “Solution to detect, classify, and report illicit online marketing and sales of controlled substances via twitter: Using machine learning and web forensics to combat digital opioid access,” J. Med. Internet Res., vol. 20, no. 4, pp. 1–13, Feb. 2018. https://doi.org/10.2196/10029
N. Shah, J. Li & T. Mackey, “An unsupervised machine learning approach for the detection and characterization of illicit drug-dealing comments and interactions on Instagram,” Subst. Abus., vol. 43, no. 1, pp. 273–277, Jul. 2022. https://doi.org/10.1080/08897077.2021.1941508
D-H. Han, S. Lee & D-C. Seo, “Using machine learning to predict opioid misuse among U.S. adolescents,” Prev. Med., vol. 130, pp. 1–10, Nov. 2019. https://doi.org/10.1016/j.ypmed.2019.105886
Z. Jianqiang, N. Chunming, P. Chengyun, H. Junxun, G. Sheng & C. Jin, “Rapid Recognition of Different Sources of Heroin Drugs by Using a Hand-Held Near-Infrared Spectrometer Based on a Multi-Layer Extreme Learning Machine Algorithm,” J. Braz. Chem. Soc., vol. 34, no. 3, pp. 426–433, Mar. 2023. https://doi.org/10.21577/0103-5053.20220120
H. Feng, R. Elladki, J. Jiang & G.-W. Wei, “Machine-learning Analysis of Opioid Use Disorder Informed by MOR, DOR, KOR, NOR and ZOR-Based Interactome Networks,” ArXiv, pp. 1–24, Jan. 2023. Available: http://arxiv.org/abs/2301.04815
J. Prieto, K. Scott, D. McEwen, L. Podewils, A. Al-Tayyib, J. Robinson, D. Edwards, S. Foldy, J. Shlay & A. Davidson, “The detection of opioid misuse and heroin use from paramedic response documentation: Machine learning for improved surveillance,” J. Med. Internet Res., vol. 22, no. 1, pp. 1–8, Jul. 2019. https://doi.org/10.2196/15645
J. Davis, P. Rao, B. Dilkina, J. Prindle, D. Eddie, N. Christie, G. DiGuiseppi, S. Saba, C. Ring & M. Dennis, “Identifying individual and environmental predictors of opioid and psychostimulant use among adolescents and young adults following outpatient treatment,” Drug Alcohol Dep., vol. 233, pp. 1–10, Apr. 2022. https://doi.org/10.1016/j.drugalcdep.2022.109359
D. Cipriano, Y. Melo, M. Zambrano, R. Ruiz y J. Deza, “A machine learning approach to find the determinants of Peruvian coca illegal crops,” Decis. Sci. Lett., vol. 11, no. 2, pp. 127–136, Dec. 2021. http://dx.doi.org/10.5267/j.dsl.2021.12.003
H. Feng, K. Gao, D. Chen, A. Robison, E. Ellsworth & G.-W. Wei, “Machine learning analysis of cocaine addiction informed by DAT, SERT, and NET-based interactome networks,” ArXiv, pp. 1–23, Jan. 2022. Available: http://arxiv.org/abs/2201.00114
K. Gao, D. Chen, A. Robison & G.-W. Wei, “Proteome-Informed Machine Learning Studies of Cocaine Addiction,” J. Phys. Chem. Lett., vol. 12, no. 45, pp. 11122–11134, Nov. 2021. https://doi.org/10.1021/acs.jpclett.1c03133
R. Suchting, J. Vincent, S. Lane, C. Green, J. Schmitz & M. Wardle, “Using a data science approach to predict cocaine use frequency from depressive symptoms,” Drug Alcohol Dep., vol. 194, pp. 310–317, Jan. 2019. https://doi.org/10.1016/j.drugalcdep.2018.10.029
R. Kranenburg, J. Verduin, Y. Weesepoel, M. Alewijn, M. Heerschop, G. Koomen, P. Keizers, F. Bakker, F. Wallace, A. van Esch, A. Hulsbergen & A. van Asten, “Rapid and robust on-scene detection of cocaine in street samples using a handheld near-infrared spectrometer and machine learning algorithms,” Drug Test Anal., vol. 12, no. 10, pp. 1404–1418, Jul. 2020. https://doi.org/10.1002/dta.2895
J. Choi, J. Chung & J. Choi, “Exploring impact of marijuana (Cannabis) abuse on adults using machine learning,” Int. J. Environ. Res. Public Health, vol. 18, no. 19, pp. 1–12. Sep. 2021. https://doi.org/10.3390/ijerph181910357
T. Parekh & F. Fahim, “Building risk prediction models for daily use of marijuana using machine learning techniques,” Drug Alcohol Dep., vol. 225, pp. 1–10, Aug. 2021. https://doi.org/10.1016/j.drugalcdep.2021.108789
D-H. Han & D-C. Seo, “Identifying risk profiles for marijuana vaping among U.S. young adults by recreational marijuana legalization status: A machine learning approach,” Drug Alcohol Dep., vol. 232, pp. 1–10, Mar. 2022. https://doi.org/10.1016/j.drugalcdep.2022.109330
L. Zoboroski, T. Wagner & B. Langhals, “Classical and neural network machine learning to determine the risk of marijuana use,” Int. J. Environ. Res. Public Health, vol. 18, no. 14, pp. 1–15. Jul. 2021. https://doi.org/10.3390/ijerph18147466
S. Negriff, B. Dilkina, L. Matai & E. Rice, “Using machine learning to determine the shared and unique risk factors for marijuana use among child-welfare versus community adolescents,” PLoS One, vol. 17, no. 9, pp. 1–19, Sept. 2022. https://doi.org/10.1371/journal.pone.0274998
N.-E. Quemá-Taimbud, M.-E. Mendoza-Becerra y O.-F. Bedoya-Leyva, “Initialization and Local Search Methods Applied to the Set Covering Problem: A Systematic Mapping,” Rev. Fac. Ing., vol. 32, no. 63, pp. 1–20, Feb. 2023. https://doi.org/10.19053/01211129.v32.n63.2023.15235
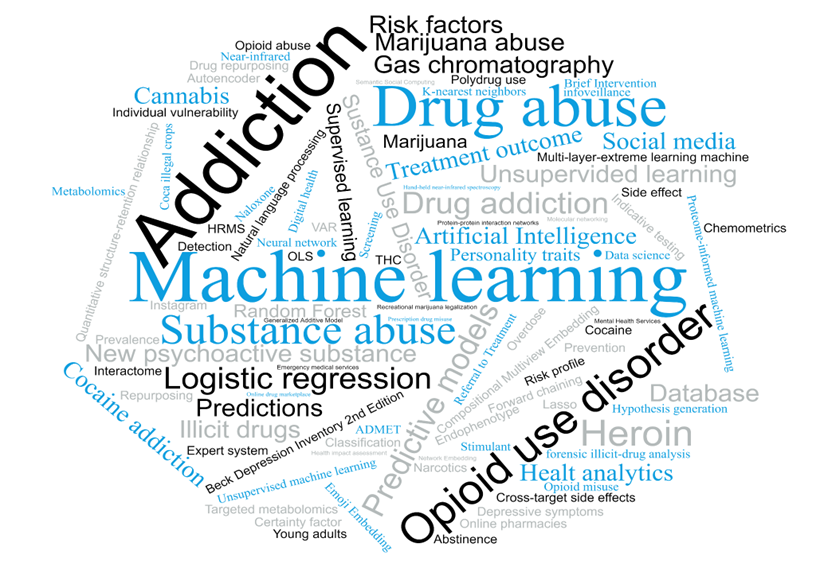
Descargas
Publicado
Cómo citar
Número
Sección
Licencia
Derechos de autor 2023 INGE CUC

Esta obra está bajo una licencia internacional Creative Commons Atribución-NoComercial-SinDerivadas 4.0.
Los artículos publicados son de exclusiva responsabilidad de sus autores y no reflejan necesariamente las opiniones del comité editorial.
La Revista INGE CUC respeta los derechos morales de sus autores, los cuales ceden al comité editorial los derechos patrimoniales del material publicado. A su vez, los autores informan que el presente trabajo es inédito y no ha sido publicado anteriormente.
Todos los artículos están bajo una Licencia Creative Commons Atribución-NoComercial-SinDerivadas 4.0 Internacional.