Modeling the probability of default and calculation of the catastrophic loss in a financial institution in Colombia
DOI:
https://doi.org/10.17981/econcuc.42.2.2021.Econ.2Keywords:
Probability of default, Logistic regression, Credit risk, Liquidity, Indebtedness, Global adjustmentAbstract
The expected loss in a financial institution is the amount of capital that would be lost as a result of the exposure that the debt has over time. This work focuses on modeling the probability of default for a loan portfolio under two study scenarios, one with a normal default level and the other with a restricted default level. A database belonging to a consumer loan portfolio is taken as a reference, with a sample of 5,000 obligations analyzed in the period from January to December 2019. The statistical method used is the logistic regression based on the financial variables of liquidity and indebtedness, plus a non-financial variable such as age. The results show a model with a global adjustment level greater than 85% in the two study scenarios, where the income variable is the one that has the most influence on the logistic regression model. Finally, the applicability of logistic regression as a statistical tool in the search for forecasting models is ratified, with which it is possible to reduce the expected loss in a loan portfolio without increasing risk exposure.
Downloads
References
Affes, Z. & Hentati, R. (2017). Predicting US Banks Bankruptcy: Logit Versus Canonical Discriminant Analysis. Computational Economics, (54), 199–244. https://doi.org/10.1007/s10614-017-9698-0
Alaka, H., Oyedele, L., Owolabi, H., Kumar, V., Ajayi, S., Akinade, O. & Bilal, M. (2018). Systematic Review of Bankruptcy Prediction Models: Towards A Framework for Tool Selection. Expert Systems With Applications, (94), 164–184. https://doi.org/10.1016/j.eswa.2017.10.040
Apilado, V., Warner, D. & Dauten, J. (1974). Evaluative Techniques in Consumer Finance-- Experimental Results and Policy Implications for Financial Institutions. The Journal Of Financial And Quantitative Analysis, 9(2), 275–283. https://doi.org/10.2307/2330105
Assef, F. & Steiner, M. (2020). Ten-year evolution on credit risk research: A systematic literature review approach and discussion. Ingenieria e Investigacion, 40(2), 1–21. https://doi.org/10.15446/ing.investig.v40n2.78649
Cappon, A., Gorenstein, A., Mignot, S. & Manuel, G. (2018). Credit ratings, default probabilities, and logarithms. Journal of Structured Finance, 24(1), 39–49. https://doi.org/10.3905/jsf.2018.24.1.039
Castro, D. y Soto, A. (2017). Estimación de la probabilidad de incumplimiento para las firmas del sector económico industrial y comercial en una entidad financiera colombiana entre los años 2009 y 2014. Cuadernos de Economía, 36(71), 293–319. https://doi.org/10.15446/ cuad.econ.v36n71.55273
Chen, N., Ribeiro, B. & Chen, A. (2016). Financial credit risk assessment: a recent review. Artificial Intelligence Review, 45(1), 1–23. https://doi.org/10.1007/s10462-015-9434-x
Davis, R., Edelman, D. & Gammerman, A. (1992). Machine learning algorithms for credit-card applications. IMA Journal of Management Mathematics, 4(1), 43–51. https://doi.org/10.1093/imaman/4.1.43
Dendramis, Y., Tzavalis, E. & Adraktas, G. (2018). Credit risk modelling under recessionary and financially distressed conditions. Journal of Banking and Finance, 91, 160–175. https://doi.org/10.1016/j.jbankfin.2017.03.020
Do Prado, J., Carvalho, F., Benedicto, G. y Lima, A. (2019). Análisis del riesgo de crédito que enfrentan las empresas de capital abierto en Brasil: un enfoque utilizando análisis discriminante regresión logística y redes neuronales artificiales. Estudios Gerenciales, 35(153), 347–360. https://doi.org/10.18046/j.estger.2019.153.3151
Durovic, A. (2017). Estimating probability of default on peer to peer market – Survival analysis approach. Journal of Central Banking Theory and Practice, 62(2), 149–167. https://doi.org/10.1515/jcbtp-2017-0017
Fantazzini, D. & Zimin, S. (2020). A multivariate approach for the simultaneous modelling of market risk and credit risk for cryptocurrencies. Journal of Industrial and Business Economics, 47(1), 19–69. https://doi.org/10.1007/s40812-019-00136-8
Grundke, P., Pliszka, K. & Tuchscherer, M. (2019). Model and estimation risk in credit risk stress tests. Review of Quantitative Finance and Accounting, 55, 163–199. https://doi.org/10.1007/s11156-019-00840-5
Imtiaz, S. & Brimicombe, A. (2017). A Better Comparison Summary of Credit Scoring Classification. International Journal of Advanced Computer Science and Applications, 8(7), 1–4. https://doi.org/10.14569/IJACSA.2017.080701
Lane, S. (1972). Submarginal Credit Risk Classification. The Journal Of Financial And Quantitative Analysis, 7(1), 1379–1385. https://doi.org/10.2307/2330069
Lohmann, C. & Ohliger, T. (2018). Nonlinear relationships in a logistic model of default for a high-default installment portfolio. Journal of Credit Risk, 14(1), 45–68. https://doi.org/10.21314/JCR.2017.232
Myers, J. & Forgy, E. (1963). The Development of Numerical Credit Evaluation Systems. Journal Of The American Statistical Association, 58(303), 799–806. https://doi.org/10.2307/2282727
RiskSimulator. (2020). [Sofware]. Available from www.software-shop.com/producto/risk-simulator
Russo, V., Lagasio, V., Brogi, M. & Fabozzi, F. (2020). Application of the Merton model to estimate the probability of breaching the capital requirements under Basel III rules. Annals of Finance, 16(1), 141–157. https://doi.org/10.1007/s10436-020-00358-0
Sariev, E. & Germano, G. (2020). Bayesian regularized artificial neural networks for the estimation of the probability of default. Quantitative Finance, 20(2), 311–328. https://doi.org/10.1080/14697688.2019.1633014
Song, S., Wang, Y. & Xu, G. (2020). On the probability of default in a market with price clustering and jump risk. Mathematics and Financial Economics, 14(2), 225–247. https://doi.org/10.1007/s11579-019-00253-x
Srinivasan, V. & Kim, Y. (1987). Credit Granting: A Comparative Analysis of Classification Procedures. The Journal Of Finance, 42(3), 665–681. https://doi.org/10.2307/2328378
SPSS. (versión 24). Statistical Package for the Social Sciences. [Sofware]. Armonk: IBM. Disponible en https://www.ibm.com/support/pages/downloading-ibm-spssstatistics-24
Superfinanciera. (2002). . Circular externa 011. Bogotá, D.C.: Superfinanciera. Disponible en http://legal.legis.com.co/document/Index?obra=legcol&document=legcol_75992041c75992048a75992043f75992034e75990430a75010151f75992034
Támara, A., Villegas, G. y De Andrés, J. (2019). Una revisión sistemática de la literatura en torno a la quiebra empresarial para el período 2012-2017. Espacios, 40(4). Disponible en http://www.revistaespacios.com/a19v40n04/a19v40n04p25.pdf
Támara, A., Villegas, G., Leones, M. y Salazar, J. (2018). Modelación del riesgo de insolvencia en empresas del sector salud empleando modelos logit. Revista de métodos cuantitativos para la economía y la empresa,, 26, 128–145. Disponible en https://www.upo.es/revistas/index.php/RevMetCuant/article/view/2757
Trejo, J., Martínez, M. & Venegas, F. (2017). Credit risk management at retail in Mexico: An econometric improvement in the selection of variables and changes in their characteristics. Contaduría y Administración, 62(2), 399–418. https://doi.org/10.1016/j.cya.2017.02.006
Tsai, C. & Hung, C. (2014). Modeling credit scoring using neural network ensembles. Kybernetes, 43(7), 1114–1123. https://doi.org/10.1108/k-01-2014-0016
Van Thiel, D. & Van Raaij, W. (2019). Artificial intelligence credit risk prediction: An empirical study of analytical artificial intelligence tools for credit risk prediction in a digital era. Journal of Risk Management in Financial Institutions, 12(3), 268–286. https://doi.org/10.33423/jaf.v19i8.2622
Wiginton, J. (1980). A note on the comparison of logit and discriminant models of consumer credit behaviour. Journal of Financial and Quantitative Analysis, 15(3), 757–770. https://doi.org/10.2307/2330408
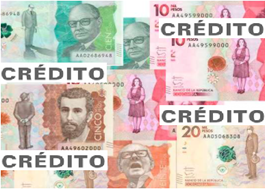
Downloads
Published
How to Cite
Issue
Section
License
Copyright (c) 2021 ECONÓMICAS CUC

This work is licensed under a Creative Commons Attribution-NonCommercial-NoDerivatives 4.0 International License.
You are free to:
- Share — copy and redistribute the material in any medium or format
- The licensor cannot revoke these freedoms as long as you follow the license terms.
Under the following terms:
- Attribution — You must give appropriate credit , provide a link to the license, and indicate if changes were made . You may do so in any reasonable manner, but not in any way that suggests the licensor endorses you or your use.
- NonCommercial — You may not use the material for commercial purposes .
- NoDerivatives — If you remix, transform, or build upon the material, you may not distribute the modified material.
- No additional restrictions — You may not apply legal terms or technological measures that legally restrict others from doing anything the license permits.