Modeling and forecasting blackberry production in Colombia using a Box Jenkins ARIMA approach
DOI:
https://doi.org/10.17981/econcuc.44.1.2023.Econ.4Keywords:
Predictive capacity, univariate analysis, data modeling, productionAbstract
Blackberry production in Colombia contributes to the nation´s gross domestic profit, employment and farmers’ social well-being. It is considered of great economic importance as blackberry fruits are used as raw material for the agroindustry. In this manner, production instability affects farmers’ economic profitability; therefore, forecasting plays an important role in monitoring production as well as in farmers´ planting decision and resource allocation. Hence, the purpose of the study was to model and forecast blackberry production in Colombia using a Box Jenkins ARIMA approach for the period 1992-2023. A quantitative, non-experimental, correlational and descriptive research design was selected. The appropriateness of the model and its predictive capacity was assessed by verifying the different goodness-of-fit criteria. Results showed that the ARIMA (1,1,0) was the most suitable model as it captured the behavior of the actual time series. Based on the forecasted values it is expected a 5.47% increase in blackberry production for the period 2021-2023 which will consequently improve farmers´ income and thus contribute to the reduction in poverty.
Downloads
References
Afzal, M., Rehman, H. U. & Butt, A. R. (2002). Forecasting: a dilemma of modules (A Comparison of Theory Based and Theory Free Approaches). Pakistan Economic and Social Review, 40(1), 1–18. Available: https://www.jstor.org/stable/25825233
Agronet. (s.f.). Blackberry statistical data [Database]. Available: http://www.agronet.gov.co/estadistica/Paginas/home.aspx
Akın, M., Eyduran, S., Çelik, Ş., Aliyev, P., Ayko, S. & Eyduran, E. (2021). Modeling and forecasting cherry production in Turkey. The Journal of Animal and Plant Science, 31(3), 773–781. https://doi.org/10.36899/JAPS.2021.3.0267
Arguello, R. & Valderrama-González, D. (2015). Sectoral and poverty impacts of agricultural policy adjustments in Colombia. Agricultural Economics, 46(2), 259–280. https://doi.org/10.1111/agec.12155
Burhan, A. & Khalid, M. (2006). Forecasting Kinnow production in Pakistan: An econometric analysis. International Journal of Agriculture & Biology, 8(4), 455–458. Available from https://www.fspublishers.org/published_papers/6916_..pdf
Brandt, J. & Bessler, D. (1984). Forecasting with Vector Autoregressions versus a Univariate ARIMA Process: An empirical example with U.S. Hog Prices. North Central Journal of Agricultural Economics, 6(2), 29–36. https://doi.org/10.2307/1349248
Brooks, C. (2019). Introductory econometrics for finance. Cambridge: Cambridge University Press. https://doi.org/10.1017/9781108524872
Cancino, S., Cancino-Escalante, G. y Cancino-Ricketts, D. (2020). Modelo econométrico para el cultivo de mora de castilla (Rubus glaucus) en los municipios de Pamplona y Chitagá, Norte de Santander, Colombia. Aibi Revista de Investigación, Administración e Ingeniería, 8(1), 37–43. https://doi.org/10.15649/2346030X.752
Cancino, S., Cancino, G. y Cancino, D. (2021). Análisis de regresión de los factores que afectan la rentabilidad económica de la producción de curuba. Dictamen Libre, (29), 1–13. https://doi.org/10.18041/2619-4244/dl.29.7861
Cárdenas M, Echavarría J, Hernández G, Maiguashca A, Meisel A, Ocampo, J. y Zárate, J. (2018). Coyuntura del sector agropecuario colombiano. [Informe de la Junta Directiva al Congreso de la República]. Bogotá, D.C.: Banco de la República. Disponible en https://www.banrep.gov.co/es/recuadro-2-coyuntura-del-sectoragropecuario-colombiano
Chen, C.-K. (2008). An integrated enrollment forecast model. AIR IR Applications, 15, 1–18. Available: https://eric.ed.gov/?id=ED504328
Dickey, D. & Fuller, W. (1979). Distribution of the estimators for autoregressive time series with a unit root. Journal of the American Statistical Association, 74(366), 427–431. https://doi.org/10.2307/2286348
E-views (version 9.0). Econometric Views. [Software]. London: IHS. Available: www.eviews.com
Franco G. y Giraldo, M. (1998). El cultivo de la mora. Mosquera: Corporación Colombiana de Investigación Agropecuaria-CORPOICA. Disponible en http://bibliotecadigital.agronet.gov.co/handle/11348/4039
FAO. (s.f.). FAOSTAT. Crops and livestock products. [Database]. Available: https://www.fao.org/faostat/en/#data/QCL
Gajbihe, S., Wankhade, R. & Mahalle, S. (2010). Forecasting chickpea production in India using ARIMA model. International Journal of Commerce and Business Management, 3(2), 212–215. Available from http://researchjournal.co.in/upload/assignments/3_212-215.pdf
Gujarati, D. y Porter, D. (2010). Econometría. México D.F.: McGraw Hill.
Hamjah, M. A. (2014). Forecasting Major Fruit Crops Productions in Bangladesh using Box-Jenkins ARIMA Model. Journal of Economics and Sustainable Development, 5(7), 96–107. Available from https://core.ac.uk/download/pdf/234646336.Pdf
Henley D. (2012). The agrarian roots of industrial growth: rural development in South-East Asia and sub-Saharan Africa. Development Policy Review, 30(51), 25–47. https://doi.org/10.1111/j.1467-7679.2012.00564.x
Iqbal, M. A. (2020). Application of regression techniques with their advantages and disadvantages. Elektor Magazine, 4(1), 11–17. Available: https://www.elektormagazine.com/magazine-archive/2020
Judge, G., Hill, R., Griffiths W., Lutkepohl, H. & Lee, T. (1991). An introduction to the theory and practice of econometrics. [2 ed.]. New York: Wiley.
Khan, S. & Khan, U. (2020). Comparison of Forecasting Performance with VAR vs. ARIMA Models Using Economic Variables of Bangladesh. Asian Journal of Probability and Statistics, 10(2), 33–47. https://doi.org/10.9734/ajpas/2020/v10i230243
Khan, D., Ullah, A., Bibi, Z., Ullah, I., Zulfiqar, M. & Khan, Z. (2020). Forecasting area and production of guava in Pakistan: An econometric analysis. Sarhad Journal of Agriculture, 36(1), 272–281. http://dx.doi.org/10.17582/journal.sja/2020/36.1.272.281
Majid, R. & Mir, S. (2018). Advances in Statistical Forecasting Methods: An Overview. Economic Affairs, 63(4), 815–831. https://doi.org/10.30954/0424-2513.4.2018.5
Mehmood, S. & Ahmad, Z. (2013). Time series model to forecast area of mangoes from Pakistan: An application of univariate ARIMA model. Academy of Contemporary Research, 2(1), 10–15.
Meyler, A., Kenny, G. & Quinn, T. (1998). Forecasting Irish inflation using ARIMA models [Technical Paper 3/RT/98]. Dublín: Central Bank of Ireland. Available: https://www.centralbank.ie/docs/default-source/publications/research-technicalpapers/3rt98---forecasting-irish-inflation-using-arima-models-(kenny-meylerand-quinn).pdf
Phillips, P. & Perron, P. (1988). Testing for a unit root in time series regression. Biometricka, 75(2), 335–346. https://doi.org/10.2307/2336182
República de Colombia. MinAgricultura. (2021). Cadena productiva de la mora Dirección de Cadenas Agrícolas y Forestales. Marzo de 2021. Bogotá, D.C.: Minagricultura.Recuperado de https://sioc.minagricultura.gov.co/Mora/Documentos/2021-03-31%20Cifras%20Sectoriales.pdf
Restrepo, M., Luna-Ramirez, J. & Castaño-Quintero, V. (2019). Evaluation of the shelf-life of blackberry pulp fortified with physiologically active compounds. Respuestas, 24(2), 16–26. Available: https://revistas.ufps.edu.co/index.php/respuestas/article/download/1827/2203?inline=1
Sánchez-López, E., Barreras-Serrano, A., Pérez-Linares, C., Figueroa-Saavedra, F. & Olivas-Valdez, J. (2013). Using an ARIMA model to forecast bovine milk production in Baja California, México. Tropical and Subtropical Agroecosystems, 16(3), 315–324. Available: https://www.revista.ccba.uady.mx/ojs/index.php/TSA/article/view/1290
Ullah, A., Khan, D. & Zheng, S. (2018). Forecasting of peach area and production wise econometric analysis. The Journal of Animal & Plant Sciences, 28(4), 1121–1127. Available: http://www.thejaps.org.pk/docs/v-28-04/23.pdf
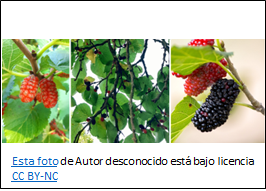
Published
How to Cite
Issue
Section
License
Copyright (c) 2023 Susan Elsa Cancino, Giovanni Orlando Cancino Escalante, Daniel Francisco Cancino Ricketts

This work is licensed under a Creative Commons Attribution-NonCommercial-NoDerivatives 4.0 International License.
You are free to:
- Share — copy and redistribute the material in any medium or format
- The licensor cannot revoke these freedoms as long as you follow the license terms.
Under the following terms:
- Attribution — You must give appropriate credit , provide a link to the license, and indicate if changes were made . You may do so in any reasonable manner, but not in any way that suggests the licensor endorses you or your use.
- NonCommercial — You may not use the material for commercial purposes .
- NoDerivatives — If you remix, transform, or build upon the material, you may not distribute the modified material.
- No additional restrictions — You may not apply legal terms or technological measures that legally restrict others from doing anything the license permits.