Valor en Riesgo y simulación: una revisión sistemática
DOI:
https://doi.org/10.17981/econcuc.43.1.2022.Econ.3Palabras clave:
Riesgo, Valor en riesgo, VaR, Bibliometría, Mapeo científicoResumen
El valor en riesgo es la medida de mercado utilizada por las instituciones financieras y adoptada por el Comité de Basilea para calcular y gestionar el riesgo, lo que la convierte en una medida necesaria para el sector financiero. En este artículo se realiza un estudio bibliométrico del Valor en Riesgo (VaR) y su cálculo mediante procesos de simulación. Para ello se revisan las investigaciones publicadas en los últimos 20 años en las bases de datos Scopus y Web of Science, recopilando los documentos más relevantes para su análisis. Posteriormente se presenta la justificación del tema y se elabora la red social utilizando la analogía del árbol, en la que cada uno de los documentos más importantes se clasifican como raíz, tronco u hoja. Finalmente, se identifican las perspectivas de investigación del tema mediante un análisis de co-citaciones. Se concluye que las mujeres tienen un alto grado de participación en cargos gerenciales, sin embargo, se nota una diferencia significativa de 3.492.556 pesos en los salarios de los dos sexos, donde los hombres son quienes obtiene mayores ingresos.
Descargas
Citas
Andersonn, F., Mausser, H., Rosen, D. & Uryasev, S. (2001). Credit risk optimization with Conditional Value-at-Risk criterion. Mathemastical Programming, 89(2), 273–291. https://doi.org/10.1007/PL00011399
Andriosopoulos, K. & Nomikos, N. (2015). Risk management in the energy markets and Value-at-Risk modelling: a hybrid approach. The European Journal of Finance, 21(7), 548–574. https://doi.org/10.1080/1351847X.2013.862173
Aria, M, y Cuccurullo, C. (2017). Bibliometrix: una herramienta R para el análisis exhaustivo de la cartografía científica. Journal of Informetrics, 11(4), 959–975. Disponible en https://doi.org/10.1016/j.joi.2017.08.007
Artzner, P., Delbaen, F., Eber, J. & Heath, D. (1999). Coherent Measures of Risk. Mathematical Finance, 9(3), 203–228. https://doi.org/10.1111/1467-9965.00068
Asimit, V., Peng, L., Wang, R. y Yu, A. (2019). An efficient approach to quantile capital allocation and sensitivity analysis. Mathematical Finance, 29(4), 1131–1156. https://doi.org/10.1111/mafi.12211
Babazadeh, H. & Esfahanipour, A. (2019). A novel multi period mean-VaR portfolio optimization model considering practical constraints and transaction cost. Journal of Computational and Applied Mathematics, 361(1), 313–342. https://doi.org/10.1016/j.cam.2018.10.039
Bastian, M., Heymann, S. & Jacomy, M. (May. 2009). Gephi: An Open Source Software for Exploring and Manipulating Networks. Presentation at Third International Conference on Weblogs and Social Media. ICWSM, San José, CA, USA. https://doi.org/10.13140/2.1.1341.1520
BIS. (2011). El Nuevo Acuerdo de Capital de Basilea. [Documento Consultivo]. Basilea: BIS. Recuperado de https://www.bis.org/publ/bcbsca03_s.pdf
Bollerslev, T. (1986). Generalized Autoregressive Conditional Heteroskedasticity. Journal of Econometrics, 31(3), 307–327. https://doi.org/10.1016/0304-4076(86)90063-1
Broadie, M., Du, Y. & Moallemi, C. C. (2011). Efficient Risk Estimation via Nested Sequential Simulation. Management Science, 57(6), 1172–1194. https://doi.org/10.1287/mnsc.1110.1330
Buitrago, S., Duque, P. y Robledo, S. (2020). Branding Corporativo: una revisión bibliográfica. Económicas CUC, 41(1), 143–162. https://doi.org/10.17981/econcuc.41.1.2020.Org.1
Chen, Q., Gerlach, R. & Lu, Z. (2012). Bayesian Value-at-Risk and expected shortfall forecasting via the asymmetric Laplace distribution. Computational Statistics and Data Analysis, 56(11), 3498–3516. https://doi.org/10.1016/j.csda.2010.06.018
Colletaz, G., Hurlin, C. & Pérignon, C. (2013). The Risk Map: A new tool for validating risk models. Journal of Banking & Finance, 37(10), 3843–3854. https://doi.org/10.1016/j.jbankfin.2013.06.006
Dahlgreen, R., Ching, C. & Lawarree, J. (2003). Risk Assessment in Energy Trading. IEEE Transactions on Power Systems, 18(2), 503–511. https://doi.org/10.1109/TPWRS.2003.810685
Du, Z. & Escanciano, J. C. (2017). Backtesting Expected Shortfall: Accounting for Tail Risk. Management Science, 63(4), 940–958. https://doi.org/10.1287/mnsc.2015.2342
Duffie, D. & Pan, J. (1997). An Overview of Value at Risk. The Journal of Derivatives. 4(3), 7–49. https://doi.org/10.3905/JOD.1997.407971
Duque, P. y Cervantes-Cervantes, L.-S. (2019). Responsabilidad Social Universitaria: una revisión sistemática y análisis bibliométrico. Estudios Gerenciales, 35(153), 451–464. https://doi.org/10.18046/j.estger.2019.153.3389
Duque, P. y Duque, E. (2020). Marketing digital y comercio electrónico: un análisis bibliométrico. En: M. Redondo, A. Barrera y C. Duque (eds.), Nuevos modelos de negocio (pp. 73–96). Pereira: Unilibre. Disponible en https://hdl.handle.net/10901/18463
Engle, R. F. (1982). Autoregressive Conditional Heteroscedasticity with Estimates of the Variance of United Kingdom Inflation. Econometrica, 50(4), 987–1007. https://doi.org/10.2307/1912773
Engle, R. F. & Manganelli, S. (2004). CAViaR: Conditional Autoregressive Value at Risk by Regression Quantiles. Journal of Business & Economic Statistics, 22,367–381. https://doi.org/10.1198/073500104000000370
Escanciano, J. C. & Pei, P. (2012). Pitfalls in backtesting Historical Simulation VaR models. Journal of Banking & Finance, 36(8), 2233–2244. https://doi.org/10.1016/j.jbankfin.2012.04.004
Gaglianone, W., Renato, L., Linton, O. & Smith, D. (2011). Evaluating Value-at-Risk Models via Quantile Regression. Journal of Business & Economic Statistics, 29(1), 150–160. https://doi.org/10.1198/jbes.2010.07318
Gaio, L., Pimenta, T., Guasti, F., Passos, I. & Oliveira, N. (2018). Value-at-risk performance in emerging and developed countries. International Journal of Managerial Finance, 14(5), 591–612. https://doi.org/10.1108/IJMF-10-2017-0244
Gephi. (versión 0.9.2). Gephi. [Visualization and exploration software for graphs and networks]. Compiègne: UTC. Available: https://gephi.org
Gencay, R. & Selcuk, F. (2004). Extreme value theory and Value-at-Risk: Relative performance in emerging markets. International Journal of Forecasting, 20(2), 287–303. https://doi.org/10.1016/j.ijforecast.2003.09.005
Gençay, R., Selçuk, F. & Ulugülyagci, A. (2003). High volatility, thick tails and extreme value theory in value-at-risk estimation. Insurance. Mathematics and Economics, 33(2), 337–356. https://doi.org/10.1016/J.INSMATHECO.2003.07.004
Glasserman, P. & Li, J. (2005). Importance Sampling for Portfolio Credit Risk. Management Science, 51(11), 1643–1656. https://doi.org/10.1287/mnsc.1050.0415
Glasserman, P., Heilderberg, P. & Shahabuddin, P. (2002). Portfolio value-at-risk with heavy-tailed risk factors. Mathematical Finance, 12(3), 239–269. https://doi.org/10.1111/1467-9965.00141
Glosten, L. R., Jagannathan, R. & Runkle, D. E. (1993). On the Relation between the Expected Value and the Volatility of the Nominal Excess. The Journal of Finance, 48(5), 1779–1801. https://doi.org/10.2307/2329067
Gordy, M. B. & Juneja, S. (2010). Nested Simulation in Portfolio Risk Measurement. Management Science, 56(10), 1833–1848. https://doi.org/10.1287/mnsc.1100.1213
Hong, L. J. (2009). Estimating Quantile Sensitivities. Operations Research, 57(1), 118–130. https://doi.org/10.1287/opre.1080.0531
Huang, D., Zhub, S., Fabozzi, F. & Fukushima, M. (2008). Portfolio selection with uncertain exit time: A robust CVaR approach. Journal of Economic Dynamics & Control, 32(2), 594–623. https://doi.org/10.1016/j.jedc.2007.03.003
Jabr, R. A. (2005). Robust Self-Scheduling Under Price Uncertainty Using Conditional Value-at-Risk. IEEE Transactions on Power Systems, 20(4), 1852–1858. https://doi.org/10.1109/TPWRS.2005.856952
Kennedy, J. & Elberhart, R. (Nov. 1995). Particle Swarm Optimization. Presented at Third International Conference on Neural Networks, ICNN, Perth, WA, Australia. https://doi.org/10.1109/ICNN.1995.488968
Kupiec, P. H. (1995). Techniques for Verifying the Accuracy of Risk Measurement Models. The Journal of Derivatives, 3(2), 73–84. https://doi.org/10.3905/JOD.1995.407942
Kuester, K., Mittnik, S. & Paoella, M. (2005). Value-at-Risk Prediction: A Comparison of Alternative Strategies. Journal of Financial Econometrics, 4(1), 53–89. https://doi.org/10.1093/jjfinec/nbj002
Liu, B. & Liu, Y.-K. (2002). Expected Value of Fuzzy Variable and Fuzzy Expected Value Models. IEEE Transactions on Fuzzy Systems, 10(4), 445–450. https://doi.org/10.1109/TFUZZ.2002.800692
Lwin, K. T., Qu, R. & MacCarthy, B. L. (2017). Mean-VaR Portfolio Optimization: A Nonparametric Approach. European Journal of Operational Research, 260(2), 751–766. https://doi.org/10.1016/j.ejor.2017.01.005
Markowitz, H. (1952). Portfolio Selection. The Journal of Finance, 7(1), 77–91. https://doi.org/10.1111/j.1540-6261.1952.tb01525.x
Marimoutou, V., Raggad, B. & Trabelsi, A. (2009). Extreme Value Theory and Value at Risk: Application to oil market. Energy Economics, 31(4), 519–530. https://doi.org/10.1016/j.eneco.2009.02.005
McNeil, A. J. & Frey, R. (2000). Estimation of tail-related risk measures for heteroscedastic financial time series: an extreme value approach. Journal of Empirical Finance, 7(3-4), 271–300. https://doi.org/10.1016/S0927-5398(00)00012-8
Moazeni, S., Coleman, T. F. & Li, Y. (2013). Smoothing and parametric rules for stochastic mean-CVaR optimal execution strategy. Annals of Operations Research, 237, 99–120. https://doi.org/10.1007/s10479-013-1391-7
Muller, F. M. & Brutti, M. (2017). Numerical comparison of multivariate models to forecasting risk measures. Risk Management, 20(1), 29–50. https://doi. org/10.1057/s41283-017-0026-8
Nelson, D. B. (1991). Conditional Heteroskedasticity in Asset Returns: A New Approach. Econometrica, 59(2), 347–370. https://doi.org/10.2307/2938260
Novales, A., & Garcia-Jorcano, L. (2018). Backtesting extreme value theory models of expected shortfall. Quantitative Finance, 19(5), 799–825. https://doi.org/10.1080/14697688.2018.1535182
Paolella, M. (2017). The Univariate Collapsing Method for Portfolio Optimization. Econometrics, 5(2), 1–33. https://doi.org/10.3390/econometrics5020018
Perignon, C. & Smith, D. (2010). The level and quality of Value-at-Risk disclosure by commercial Banks. Journal of Banking & Finance, 34(2), 362–377. https://doi.org/10.1016/J.JBANKFIN.2009.08.009
Pritsker, M. (2006). The hidden dangers of historical simulation. Journal of Banking & Finance, 30(2), 561–582. https://doi.org/10.1016/j.jbankfin.2005.04.013
R. (version 3). Statistical computing and graphics. [Software environment]. Vienna: R Core Team. Available: https://www.r-project.org/
Robledo, S., Osorio, G. y López, C. (2014). Networking en pequeña empresa: una revisión bibliográfica utilizando la teoria de grafos. Revista Vínculos, 11(2), 117–134. https://revistas.udistrital.edu.co/index.php/vinculos/article/view/9664
Rockafellar, R. T. & Uryasev, S. (2000). Optimization of conditional value-at-risk. Risk.net, 2(3), 21–41. https://doi.org/10.21314/JOR.2000.038
Scheller, F. & Auer, B. (2018). How does the choice of Value-at-Risk estimator influence asset allocation decisions? Quantitative Finance, 18(12), 2005–2022. https://doi.org/10.1080/14697688.2018.1459806
Sci2 Tool. (versión 1.3). Science of Science. [Software]. Bloomington: CNS. Available: https://sci2.cns.iu.edu/user/index.php
Vijverberg, C.-P. C., Vijverberg, W. P. M. & Taşpınar, S. (2015). Linking Tukey’s Legacy to Financial Risk Measurement. Computational Statistics and Data Analysis, 100, 595–615. https://doi.org/10.1016/j.csda.2015.08.018
Wasserman, S. & Faust, K. (1994). Social Network Analysis: Methods and Applications. Cambridge: CUP. https://doi.org/10.1017/CBO9780511815478
Wang, B., Wang, S. & Watadata, J. (2011). Fuzzy-Portfolio-Selection Models With Value-at-Risk. IEEE Transactions on Fuzzy Systems, 19(4), 758–769. https://
doi.org/10.1109/TFUZZ.2011.2144599
Wang, C., Chen, Q. & Gerlach, R. (2018). Bayesian realized-GARCH models for financial tail risk forecasting incorporating the two-sided Weibull distribution. Quantitative Finance, 19(6), 1017–1042. https://doi.org/10.1080/14697688.2018.1540880
Xu, L. (2014). Model-free inference for tail risk measures. Econometric Theory, 34(3), 1–32. https://doi.org/10.1017/S0266466614000802
Yao, H., Li, Z. & Lai, Y. (2013). Mean–CVaR portfolio selection: A nonparametric estimation framework. Computers & Operations Research, 40(4), 1014–1044. https://doi.org/10.1016/J.COR.2012.11.007
Zaichao, Du. & Escanciano, J. C. (2016). Backtesting Expected Shortfall: Accounting for Tail Risk. Management Science, 63(4), 940–958. https://doi.org/10.1287/mnsc.2015.2342
Zhang, L., Luo, M. & Boncella, R. (2017). Product information diffusion in a social network. Electronic Commerce Research, 20(1), 3–19. https://doi.org/10.1007/s10660-018-9316-9
Zhang, Q. & Wang, X. (2009). Hedge Contract Characterization and Risk-Constrained Electricity Procurement. IEEE Transactions on Power Systems, 24(3), 1547–1548. https://doi.org/10.1109/TPWRS.2009.2021233
Ziggel, D., Berens, T., Weib, G. & Wied, D. (2014). A new set of improved Valueat-Risk back tests. Journal of Banking & Finance, 48(C), 29–41. https://doi.org/10.1016/J.JBANKFIN.2014.07.005
Zuluaga, M., Robledo, S., Osorio, G. A., Yathe, L., González, D. & Taborda, G. (2016). Metabolómica y Pesticidas: Revisión sistemática de literatura usando teoría de grafos para el análisis de referencias. Nova, 14(25), 121–128. https://doi.org/10.22490/24629448.1735
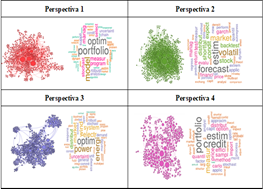
Publicado
Cómo citar
Número
Sección
Licencia
Derechos de autor 2021 ECONÓMICAS CUC

Esta obra está bajo una licencia internacional Creative Commons Atribución-NoComercial-SinDerivadas 4.0.
Usted es libre de:
- Compartir — copiar y redistribuir el material en cualquier medio o formato
- La licenciante no puede revocar estas libertades en tanto usted siga los términos de la licencia
Bajo los siguientes términos:
- Atribución — Usted debe dar crédito de manera adecuada , brindar un enlace a la licencia, e indicar si se han realizado cambios . Puede hacerlo en cualquier forma razonable, pero no de forma tal que sugiera que usted o su uso tienen el apoyo de la licenciante.
- NoComercial — Usted no puede hacer uso del material con propósitos comerciales .
- SinDerivadas — Si remezcla, transforma o crea a partir del material, no podrá distribuir el material modificado.
- No hay restricciones adicionales — No puede aplicar términos legales ni medidas tecnológicas que restrinjan legalmente a otras a hacer cualquier uso permitido por la licencia.