Penalised regressions vs. autoregressive moving average models for forecasting inflation
DOI:
https://doi.org/10.17981/econcuc.41.1.2020.Econ.3Palabras clave:
Regresión de arista, Modelo lineal penalizado, ARMA, SARMA, Pronóstico de la inflaciónResumen
This paper relates seasonal autoregressive moving average (SARMA) models with linear regression. Based on this relation, the paper shows that penalised linear models may surpass the out-of-sample forecasting accuracy of the best SARMA models when forecasting inflation based on past values, due to penalisation and cross-validation. The paper constructs a minimal working example using ridge regression to compare both of the competing approaches when forecasting the monthly inflation in 35 selected countries of the Organisation for Economic Co-operation and Development and in three groups of countries. The results empirically verify the hypothesis that penalised linear regression, and ridge regression in particular, can outperform the best standard SARMA models computed through a grid search when forecasting inflation. Thus, a new and effective technique for forecasting inflation based on past values is provided for use by financial analysts and investors. The results indicate that more attention should be given to machine learning techniques for time series forecasting of inflation, even as basic as penalised linear regressions, due to their superior empirical performance.
Descargas
Citas
Anzola, C., Vargas, P. & Morales, A. (2019). Transición entre sistemas financieros bancarios y bursátiles. Una aproximación mediante modelo de Swithing Markov. Económicas CUC, 40(1), 123–144. https://doi.org/10.17981/econcuc.40.1.2019.08
Box, G. E. P. & Jenkin, G. M. (1976). Time series analysis, forecasting and control. San Francisco: Holden-Day.
Burnham, K. P. & Anderson, D. R. (2004). Multimodel inference. Sociological Methods & Research, 33(2), 261–304. https://doi.org/10.1177/0049124104268644
Dickey, D. A., & Fuller, W. A. (1979). Distribution of the estimators for autoregressive time series with a unit root. Journal of the American Statistical Association, 74(366), 427–431. https://doi.org/10.2307/2286348
Diebold, F. X. & Mariano, R. S. (2002). Comparing Predictive Accuracy. Journal of Business & Economic Statistics, 20(1), 134–144. https://doi.org/10.1198/073500102753410444
Faust, J. & Wright, J. H. (2013). Forecasting Inflation. In, G. Elliott & A. Timmermann (Eds.), Handbook of Economic Forecasting, Vol. 2. Part. A (pp. 2–56). Amsterdam: Elsevier. https://doi.org/10.1016/B978-0-444-53683-9.00001-3
Gil, J., Castellanos, D. & Gonzalez, D. (2019). Margen de intermediación y concentración bancaria en Colombia: un análisis para el periodo 2000-2017. Económicas CUC, 40(2), 9–30. https://doi.org/10.17981/econcuc.40.2.2019.01
Gómez, C., Sánchez, V. & Millán, E. (2019). Capitalismo y ética: una relación de tensiones. Económicas CUC, 40(2), 31–42. https://doi.org/10.17981/econcuc.40.2.2019.02
Gu, S., Kelly, B. T. & Xiu, D. (december, 2018). Empirical Asset Pricing Via Machine Learning [Paper 18-04]. 31st Australasian Finance and Banking Conference, AFBC, Sydney, Australia, 1–79. https://doi.org/10.2139/ssrn.3159577
Hoerl, A. E. & Kennard, R. W. (1970). Ridge Regression: Biased Estimation for Nonorthogonal Problems. Technometrics, 12(1), 55–67. https://doi.org/10.2307/1267351
Hyndman, R. J. (july, 2013). Facts and fallacies of the AIC. [Online]. Available from https://robjhyndman.com/hyndsight/aic/
Hyndman, R. J. & Khandakar, Y. (2008). Automatic Time Series Forecasting: The forecast Package for R. Journal of Statistical Software, 27(1), 1–22. https://doi.org/10.18637/jss.v027.i03
Kvalseth, T. O. (1985). Cautionary Note about R 2. The American Statistician, 39(4), 279–285. https://doi.org/10.2307/2683704
MacKinnon, J. G. (1996). Numerical distribution functions for unit root and cointegration tests. Journal of Applied Econometrics, 11(6), 601–618. https://doi.org/10.1002/(SICI)1099-1255(199611)11:6<601::AID-JAE417>3.0.CO;2-T
Mockus, J. (1989). Bayesian approach to global optimization. Dordrecht: Kluwer Academic Publishers.
Mullainathan, S. & Spiess, J. (2017). Machine Learning: An Applied Econometric Approach. Journal of Economic Perspectives, 31(2), 87–106. https://doi.org/10.1257/jep.31.2.87
OECD. (2019). Inflation (CPI). [indicator]. https://doi.org/10.1787/eee82e6e-en
Osborn, D. R., Chui, A. P. L., Smith, J. P. & Birchenhall, C. R. (2009). Seasonality and the order of integration for consumption. Oxford Bulletin of Economics and Statistics, 50(4), 361–377. https://doi.org/10.1111/j.1468-0084.1988.mp50004002.x
Quinn, T., Kenny, G. & Meyler, A. (1999). Inflation analysis: An overview. [MPRA Paper No. 11361]. Munich: UTC. Retrieved from https://mpra.ub.uni-muenchen.de/11361/1/MPRA_paper_11361.pdf
Santosa, F. & Symes, W. W. (1986). Linear Inversion of Band-Limited Reflection Seismograms. SIAM Journal on Scientific and Statistical Computing, 7(4), 1307–1330. https://doi.org/10.1137/0907087
Tibshirani, R. (1996). Regression Shrinkage and Selection Via the Lasso. Journal of the Royal Statistical Society: Series B (Methodological), 58(1), 267–288. https://doi.org/10.1111/j.2517-6161.1996.tb02080.x
Tikhonov, A. N. & Arsenin, V. Y. (1977). Solution of ill-posed problems. Washington: Winston & Sons.
Zou, H. & Hastie, T. (2005). Regularization and variable selection via the elastic net. Journal of the Royal Statistical Society: Series B (Statistical Methodology), 67(2), 301–320. https://doi.org/10.1111/j.1467-9868.2005.00503.x
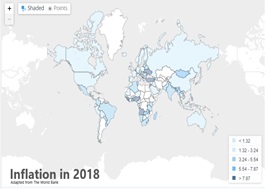
Publicado
Cómo citar
Número
Sección
Licencia
Derechos de autor 2020 Javier Humberto Ospina-Holguín, Ana Milena Ospina-Holguín

Esta obra está bajo una licencia internacional Creative Commons Atribución-NoComercial-SinDerivadas 4.0.
Usted es libre de:
- Compartir — copiar y redistribuir el material en cualquier medio o formato
- La licenciante no puede revocar estas libertades en tanto usted siga los términos de la licencia
Bajo los siguientes términos:
- Atribución — Usted debe dar crédito de manera adecuada , brindar un enlace a la licencia, e indicar si se han realizado cambios . Puede hacerlo en cualquier forma razonable, pero no de forma tal que sugiera que usted o su uso tienen el apoyo de la licenciante.
- NoComercial — Usted no puede hacer uso del material con propósitos comerciales .
- SinDerivadas — Si remezcla, transforma o crea a partir del material, no podrá distribuir el material modificado.
- No hay restricciones adicionales — No puede aplicar términos legales ni medidas tecnológicas que restrinjan legalmente a otras a hacer cualquier uso permitido por la licencia.