Ferramentas Tecnológicas Baseadas em Inteligência Artificial na Indústria Açucareira: Uma Análise Bibliométrica e Perspectivas Futuras para Eficiência Energética
DOI:
https://doi.org/10.17981/ladee.04.02.2023.4Palavras-chave:
Avanços tecnológicos, indústria açucareira, inteligência artificialResumo
Introdução: A aplicação da Inteligência Artificial –IA– na produção industrial de açúcar, particularmente em dados de sensores e gestão de sistemas, está a evoluir rapidamente para programas de monitorização em tempo real que oferecem recomendações valiosas e apoio à tomada de decisões na indústria açucareira. Metodologia: Esta análise bibliométrica abrangente de 125 artigos indexados pela Scopus destaca tendências significativas na área, incluindo aumentos na produção de artigos durante 2017, 2018, 2021 e 2022, representando 34% do total de publicações. Resultados: A produção científica neste domínio cresceu 3,93% entre 1969 e 2023. A maior parte da investigação (81%) teve origem em países-chave, incluindo Austrália, Brasil, Índia, China, Filipinas, Estados Unidos e França. Periódicos proeminentes desempenharam um papel fundamental, representando 19% das publicações. Autores notáveis incluem Attard, Everingham, Meng e Sexton, com quatro artigos publicados cada. Notavelmente, 88% dos investigadores nesta área são transitórios. Este estudo ressalta o crescimento dinâmico das aplicações de inteligência artificial na produção de açúcar, enfatizando a sustentabilidade na gestão de dados e sistemas. Conclusões: A integração eficaz destas tecnologias pode melhorar as práticas de sustentabilidade, otimizando a eficiência e a qualidade em toda a cadeia de abastecimento da produção de açúcar, contribuindo para a concretização do Objetivo de Desenvolvimento Sustentável 9. Isto porque a utilização da inteligência artificial para otimizar a produção industrial de açúcar representa uma inovação tecnológica que pode melhorar a eficiência e a infraestrutura da indústria açucareira. Isto, por sua vez, pode contribuir para alcançar o desenvolvimento sustentável à escala global.
Downloads
Referências
Andreo-Martínez, P., Ortiz-Martínez, V. M., García-Martínez, N., López, P. P., Quesada-Medina, J., Cámara, M. Á. & Oliva, J. (2020). A descriptive bibliometric study on the bioavailability of pesticides in vegetables, food, or wine research (1976–2018). Environmental Toxicology and Pharmacology, 77(103374), 103374. https://doi.org/10.1016/j.etap.2020.103374
Badshah, M., Lam, D. M., Liu, J. & Mattiasson, B. (2012). Use of an Automatic Methane Potential Test System for evaluating the biomethane potential of sugarcane bagasse after different treatments. Bioresource Technology, 114, 262–269. https://doi.org/10.1016/j.biortech.2012.02.022
Barbosa, R. M., Batista, B. L., Barião, C. V., Varrique, R. M., Coelho, V. A., Campiglia, A. D. & Barbos, F. (2015). Simple and practical control of the authenticity of organic sugarcane samples based on the use of machine-learning algorithms and trace elements determination by inductively coupled plasma mass spectrometry. Food Chemistry, 184, 154–159. https://doi.org/10.1016/j.foodchem.2015.02.146
Barrera, K. V., Pinzón, J. S., Acuña, J. S. & Jiménez-Barbosa, W. G. (2021). Bibliometric analysis of scientific journals related to optometry in Colombia (2014-2019). Revista Salud Bosque, 11(1), 1–20. https://doi.org/10.18270/rsb.v11i1.3412
Bocca, F. F. & Rodrigues, L. (2016). The effect of tuning, feature engineering, and feature selection in data mining applied to rainfed sugarcane yield modeling. Computers and Electronics in Agriculture, 128, 67–76. https://doi.org/10.1016/j.compag.2016.08.015
Brett, P., McCarthy, A., McCarthy, C., Long, D., Gillies, M., Foley, J. & Baillie, C. (2019, 30 April - 3 May). Advancing automation in the agricultural working environment [Conference]. 41st Annual Conference - Australian Society of Sugar Cane Technologists, ASSCT, Toowoomba, Queensland, Australia. https://www.assct.com.au/conference/past-conferences/172-2019-assct-conference
Chia, M. Y., Huang, Y. F., Koo, C. H. & Fung, K. F. (2020). Recent advances in evapotranspiration estimation using artificial intelligence approaches with a focus on hybridization techniques—a review. Agronomy, 10(1), 1–33. https://doi.org/10.3390/agronomy10010101
De Souza, C. H., Lamparelli, R. A., Rocha, J. & Magalhães, P. S. (2017). Mapping skips in sugarcane fields using object-based analysis of unmanned aerial vehicle (UAV) images. Computers and Electronics in Agriculture, 143, 49–56. https://doi.org/10.1016/j.compag.2017.10.006
Dongre, V. B. & Gandhi, R. S. (2016). Applications of artificial neural networks for enhanced livestock productivity: a review. Indian Journal of Animal Sciences, 86(11), 1232–1237.
El Hajj, M., Bégué, A., Guillaume, S. & Martiné, J.-F. (2009). Integrating SPOT-5 time series, crop growth modeling, and expert knowledge for monitoring agricultural practices — The case of sugarcane harvest on Reunion Island. Remote Sensing of Environment, 113(10), 2052–2061. https://doi.org/10.1016/j.rse.2009.04.009
Eli-Chukwu, N. C. (2019). Applications of artificial intelligence in agriculture: A review. Engineering, Technology & Applied Science Research, 9(4), 4377–4383. https://doi.org/10.48084/etasr.2756
Evans, K. J., Terhorst, A. & Kang, B. H. (2017). From data to decisions: helping crop producers build their actionable knowledge. Critical reviews in plant sciences, 36(2), 71–88. https://doi.org/10.1080/07352689.2017.1336047
Gillies, M., Attard, S., Jaramillo, A., Davis, M. & Foley, J. (2017, 3-5 May). Smart automation of furrow irrigation in the sugar industry [Conference]. 39th Conference of the Australian Society of Sugar Cane Technologists, ASSCT, Cairns City, Australia. https://www.assct.com.au/conference/past-conferences/112-2017-assct-conference
Godoi, A. F. L., Ravindra, K., Godoi, R. H. M., Andrade, S. J., Santiago-Silva, M., Van Vaeck, L. & Van Grieken, R. (2004). Fast chromatographic determination of polycyclic aromatic hydrocarbons in aerosol samples from sugar cane burning. Journal of Chromatography A, 1027(1–2), 49–53. https://doi.org/10.1016/j.chroma.2003.10.048
Goswami, L., Kayalvizhi, R., Dikshit, P. K., Sherpa, K. C., Roy, S., Kushwaha, A., Kim, B. S., Banerjee, R., Jacob, S. & Rajak, R. C. (2022). A critical review on prospects of bio-refinery products from second and third-generation biomasses. Chemical Engineering Journal, 448, 137677. https://doi.org/10.1016/j.cej.2022.137677
Gozá, O., De Alejo, H. E. & Rijckaert, M. (2002). Use of Simulation and Expert Systems to increase the energy efficiency in cane sugar factories. Developments in Chemical Engineering and Mineral Processing, 10(1-2), 165–179. https://dx.doi.org/10.1002/apj.5500100112
Ihaka, R. & Gentleman, R. (2023). R (version 4.3.1). R Development Core Team. https://www.r-project.org/
Iturralde, L. A., Bambi, E. & Espinosa, A. R. (2021). Methodology for sugar energy balance of power stations. LADEE, 2(2), 1–15. https://doi.org/10.17981/ladee.02.02.2022.01
Jha, K., Doshi, A., Patel, P. & Shah, M. (2019). A comprehensive review on automation in agriculture using artificial intelligence. Artificial Intelligence in Agriculture, 2, 1–12. https://doi.org/10.1016/j.aiia.2019.05.004
Jonker, J. G. G., van der Hilst, F., Junginger, H. M., Cavalett, O., Chagas, M. F. & Faaij, A. P. C. (2015). Outlook for ethanol production costs in Brazil up to 2030, for different biomass crops and industrial technologies. Applied Energy, 147, 593–610. https://doi.org/10.1016/j.apenergy.2015.01.090
Kaab, A., Sharifi, M., Mobli, H., Nabavi-Pelesaraei, A. & Chau, K.-W. (2019). Combined life cycle assessment and artificial intelligence for prediction of output energy and environmental impacts of sugarcane production. The Science of the Total Environment, 664, 1005–1019. https://doi.org/10.1016/j.scitotenv.2019.02.004
Kakani, V., Nguyen, V. H., Kumar, B. P., Kim, H. & Pasupuleti, V. R. (2020). A critical review on computer vision and artificial intelligence in food industry. Journal of Agriculture and Food Research, 2, 100033. https://doi.org/10.1016/j.jafr.2020.100033
Kantasa-Ard, A., Bekrar, A., Ait El Cad, A. & Sallez, Y. (2019). Artificial intelligence for forecasting in supply chain management: a case study of White Sugar consumption rate in Thailand. IFAC-PapersOnLine, 52(13), 725–730. https://doi.org/10.1016/j.ifacol.2019.11.201
Kapoor, M., Panwar, D. & Kaira, G. S. (2016). Bioprocesses for enzyme production using Agro-industrial wastes. In G. S. Dhillon and S. Kaur, Agro-Industrial Wastes as Feedstock for Enzyme Production (pp. 61–93). Elsevier. https://doi.org/10.1016/B978-0-12-802392-1.00003-4
Karri, R. R., Sahu, J. N. & Meikap, B. C. (2020). Improving the efficacy of the Cr (VI) adsorption process on sustainable adsorbent derived from waste biomass (sugarcane bagasse) with the help of ant colony optimization. Industrial Crops and Products, 143(111927), 111927. https://doi.org/10.1016/j.indcrop.2019.111927
Koulouris, A., Misailidis, N. & Petrides, D. (2021). Applications of process and digital twin models for production simulation and scheduling in the manufacturing of food ingredients and products. Food and Bioproducts Processing, 126, 317–333. https://doi.org/10.1016/j.fbp.2021.01.016
Le Blond, J. S., Williamson, B. J., Horwell, C. J., Monro, A. K., Kirk, C. A. & Oppenheimer, C. (2008). Production of potentially hazardous respirable silica airborne particulate from the burning of sugarcane. Atmospheric Environment, 42(22), 5558–5568. https://doi.org/10.1016/j.atmosenv.2008.03.018
Leidien University. (2019). VOSviewer (version 1.6.19). https://www.vosviewer.com/
Luciano, A. C., Picoli, M. C., Rocha, J., Franco, H., Sanches, G., Leal, M., & Le Maire, G. (2018). Generalized space-time classifiers for monitoring sugarcane areas in Brazil. Remote sensing of environment, 215, 438–451. https://doi.org/10.1016/j.rse.2018.06.017
Maldaner, F. L., Corrêdo, L., Canata, T. F. & Molin, J. P. (2021). Predicting the sugarcane yield in real-time by harvester engine parameters and machine learning approaches. Computers and Electronics in Agriculture, 181(105945), 105945. https://doi.org/10.1016/j.compag.2020.105945
Martín-del-Río, B., Neipp, M.-C., García-Selva, A. & Solanes-Puchol, A. (2021). Positive organizational psychology: A bibliometric review and science mapping analysis. International Journal of Environmental Research and Public Health, 18(10), 1–17. https://doi.org/10.3390/ijerph18105222
Muir, A., Harrison, E. & Wheals, A. (2011). A multiplex set of species-specific primers for rapid identification of members of the genus Saccharomyces. FEMS yeast research, 11(7), 552–563. https://doi.org/10.1111/j.1567-1364.2011.00745.x
Natarajan, R., Subramanian, J. & Papageorgiou, E. I. (2016). Hybrid learning of fuzzy cognitive maps for sugarcane yield classification. Computers and Electronics in Agriculture, 127, 147–157. https://doi.org/10.1016/j.compag.2016.05.016
Patrício, D. I. & Rieder, R. (2018). Computer vision and artificial intelligence in precision agriculture for grain crops: A systematic review. Computers and electronics in agriculture, 153, 69–81. https://doi.org/10.1016/j.compag.2018.08.001
Ridge, R. (2003). Trends in sugar cane mechanization. International Sugar Journal, 105(1252), 150–154. https://internationalsugarjournal.com/
Rivera, E., Rabelo, S., Garcia, D., Maciel Filho, R. & da Costa, A. (2010). Enzymatic hydrolysis of sugarcane bagasse for bioethanol production: determining optimal enzyme loading using neural networks. Journal of Chemical Technology and Biotechnology, 85(7), 983–992. https://doi.org/10.1002/jctb.2391
Rivera, E., Costa, A., Andrade, R., Atala, D., Maugeri, F. & Filho, R. (2007). Development of adaptive modeling techniques to describe the temperature-dependent kinetics of biotechnological processes. Biochemical Engineering Journal, 36(2), 157–166. https://doi.org/10.1016/j.bej.2007.02.011
Rooh, U. A., Li, A., & Ali, M. M. (2015). Fuzzy, neural network and expert systems methodologies and applications-a review. Journal of Mobile Multimedia, 11(1-2), 157–176. https://dl.acm.org/doi/abs/10.5555/2871240.2871253
Shaikh, T. A., Rasool, T. & Lone, F. R. (2022). Towards leveraging the role of machine learning and artificial intelligence in precision agriculture and smart farming. Computers and Electronics in Agriculture, 198(C), 107119. https://doi.org/10.1016/j.compag.2022.107119
Solarte-Toro, J. C. & Cardona, C. A. (2023). Sustainability of Biorefineries: Challenges and Perspectives. Energies, 16(9), 1–29. https://doi.org/10.3390/en16093786
Stuurman, K. & Lachaud, E. (2022). Regulating AI. A label to complete the proposed Act on Artificial Intelligence. Computer Law & Security Review, 44, 1–23. https://doi.org/10.1016/j.clsr.2022.105657
Stray, B. J., van Vuuren, J. H. & Bezuidenhout, C. N. (2012). An optimization-based seasonal sugarcane harvest scheduling decision support system for commercial growers in South Africa. Computers and Electronics in Agriculture, 83, 21–31. https://doi.org/10.1016/j.compag.2012.01.009
Talaviya, T., Shah, D., Patel, N., Yagnik, H. & Shah, M. (2020). Implementation of artificial intelligence in agriculture for optimization of irrigation and application of pesticides and herbicides. Artificial Intelligence in Agriculture, 4, 58–73. https://doi.org/10.1016/j.aiia.2020.04.002
Verdouw, C. N., Wolfert, J., Beulens, A. J. M. & Rialland, A. (2016). Virtualization of food supply chains with the Internet of Things. Journal of Food Engineering, 176, 128–136. https://doi.org/10.1016/j.jfoodeng.2015.11.009
Vieira, M. A., Formaggio, A. R., Rennó, C. D., Atzberger, C., Aguiar, D. A. & Mello, M. P. (2012). Object-Based Image Analysis and Data Mining applied to a remotely sensed Landsat time series to map sugarcane over large areas. Remote Sensing of Environment, 123, 553–562. https://doi.org/10.1016/j.rse.2012.04.011
Vitcosque, G. L., Fonseca, R. F., Rodríguez-Zúñiga, U. F., Bertucci.Neto, V., Couri, S. & Farinas, C. S. (2012). Production of biomass-degrading multienzyme complexes under solid-state fermentation of soybean meal using a bioreactor. Enzyme Research, 1–10. https://doi.org/10.1155/2012/248983
Wang, E., Attard, S., Everingham, Y., Philippa, B. & Xiang, W. (2018, 18-20 April). Smarter irrigation management in the sugarcane farming system using the Internet of Things [Conference]. 40th Annual Conference Australian Society of Sugar Cane Technologists, ASSCT, Mackay, Australia. https://www.assct.com.au/conference/past-conferences/153-2018-assct-conference
Wang, E., Attard, S., Linton, A., McGlinchey, M., Xiang, W., Philippa, B. & Everingham, Y. (2020). Development of a closed-loop irrigation system for sugarcane farms using the Internet of Things. Computers and Electronics in Agriculture, 172(105376), 105376. https://doi.org/10.1016/j.compag.2020.105376
West, N. (2021, 20-23 April). Management of the technical skillset required to support automation in the sugar industry [Conference]. 42nd Australian Society of Sugar Cane Technologists Conference 2021, ASSCT, Bundaberg, Australia. https://www.proceedings.com/australian-society-of-sugar-cane-technologists-assct/
Yoosefzadeh-Najafabadi, M., Hesami, M. & Eskandari, M. (2023). Machine learning-assisted approaches in modernized plant breeding programs. Genes, 14(4), 1–22. https://doi.org/10.3390/genes14040777
Zafar, M., Kumar, S., Kumar, S. & Dhiman, A. K. (2012). Artificial intelligence-based modeling and optimization of poly(3-hydroxybutyrate-co-3-hydroxyvalerate) production process by using Azohydromonas lata MTCC 2311 from cane molasses supplemented with volatile fatty acids: A genetic algorithm paradigm. Bioresource Technology, 104, 631–641. https://doi.org/10.1016/j.biortech.2011.10.024
Zhang, J., Meng, Y., Wu, J., Qin, J., Wang, H., Yao, T. & Yu, S. (2020). Monitoring sugar crystallization with deep neural networks. Journal of Food Engineering, 280(109965), 109965. https://doi.org/10.1016/j.jfoodeng.2020.109965.
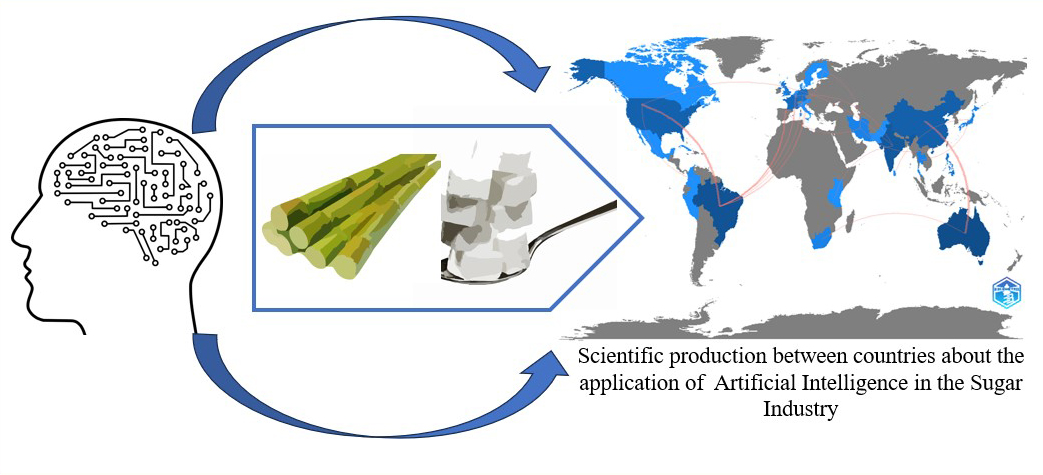
Downloads
Publicado
Como Citar
Edição
Secção
Licença
Direitos de Autor (c) 2023 Hugo Gaspar Hernandez-Palma, Jonny Rafael Plaza Alvarado, Jesús Enrique García Guiliany, Andrea Liliana Moreno Rios

Este trabalho encontra-se publicado com a Licença Internacional Creative Commons Atribuição-NãoComercial-SemDerivações 4.0.
Você tem o direito de:
- Compartilhar — copiar e redistribuir o material em qualquer suporte ou formato
- O licenciante não pode revogar estes direitos desde que você respeite os termos da licença.
De acordo com os termos seguintes:
- Atribuição — Você deve atribuir o devido crédito , fornecer um link para a licença, e indicar se foram feitas alterações . Você pode fazê-lo de qualquer forma razoável, mas não de uma forma que sugira que o licenciante o apoia ou aprova o seu uso.
- NãoComercial — Você não pode usar o material para fins comerciais .
- SemDerivações — Se você remixar, transformar, ou criar a partir do material, não pode distribuir o material modificado.
- Sem restrições adicionais — Você não pode aplicar termos jurídicos ou medidas de caráter tecnológico que restrinjam legalmente outros de fazerem algo que a licença permita.